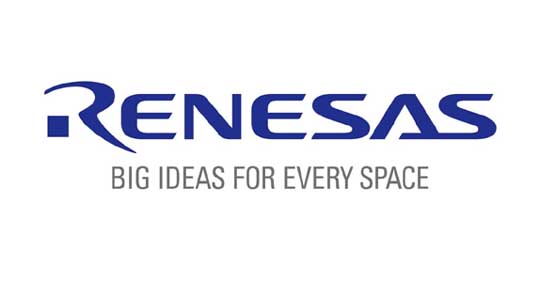
Renesas Partners with Tata to Accelerate Progress in Advanced Electronics
TOKYO― Renesas Electronics Corporation has announced a strategic partnership with Tata Motors Ltd. (TML) and Tejas Networks Ltd. (Tejas), both Tata

No patents for AnotherBrain but there are a few for Another Brain.
https://worldwide.espacenet.com/patent/search/family/069024225/publication/WO2021058466A1?q=pa = "another brain"
Maisonnier: this seems to be one of his last inventions for Softbank Robotics:
US10481270B2 Device for detecting an obstacle by means of intersecting planes and detection method using such a device
The nearest it gets to NNs is a reference to "analytic means".
A bit of background on Maisonnier:
https://robohub.org/bruno-maisonnier-moves-on-from-aldebaran/
Bruno Maisonnier moves on from Aldebaran
20150223
Farewell and thank you to Bruno Maisonnier, the founder and CEO of Aldebaran Robotics, which is now 95% owned by Softbank. Softbank had previously acquired a majority stake in the ground breaking French robotics company which created the humanoid NAO. Together Aldebaran and Softbank Robotics developed Pepper, the ‘world’s first robot that reads emotions’. Pepper is on display in Softbank and Nestle stores in Japan and there are somewhat delayed plans for Pepper’s sale in the US later in 2015.
...
Maisonnier has agreed to sell his remaining shares in Aldebaran, step down as CEO and will become a Special Advisor to Masayoshi Son, the President of Softbank Corp. In a press release from Aldebaran, February 23rd, Maisonnier says that he wants to step back from the day to day operations of growing a robotics company and reflect on the changes that have occurred in the world, analyzing and integrating them back into the world of robotics.
WO2021156271A1 ANOMALY DETECTOR, METHOD OF ANOMALY DETECTION AND METHOD OF TRAINING AN ANOMALY DETECTOR
Priority: 20200205
View attachment 10588
1. A method of constructing an anomaly detector for detecting an anomaly in a digital sample of a predetermined type and predetermined first resolution, said method comprising:
- exposing a teacher neural network trained to extract features from digital data sets, to a plurality of digital samples of a training dataset of said predetermined type, to extract features representing each said digital sample at one or more levels;
- exposing an auto-encoder to each said digital sample to reconstruct features representing said digital sample at one or more levels;
- determining a difference value reflecting the difference between said extracted features and respective said reconstructed features for each said sample; and
- repeating said steps of reconstructing features representing said training dataset with further said parameters until a minimal said difference value is obtained across said training dataset.
An anomaly detector uses two neural networks, the first, a general purpose classifying convolutional neural network operates as a teacher neural network, while a second neural network in an auto-encoder type configuration. Each of the two neural networks receives the same input stream, and generates respective feature outputs at different levels, corresponding to different resolutions for image data. The respective outputs of the two neural networks are compared at each level, and the resulting difference values consolidated across the difference levels to obtain a final difference value. In a training phase this difference value is used to drive the determination of the weights and biases of the auto-encoder, so as to obtain a auto-encoder trained for a particular input type, under the influence of the teacher neural network. In an operational mode, the difference value is compared to a threshold to determine whether a particular sample is anomalous or not. In certain embodiments, difference values a different levels may be scaled so as to be superimposed at a common resolution, thereby providing an error map indicating the location of anomalous values across the sample.
Solid state LiDaR, no moving parts, electronically steered beam.I am seriously surprised! Startup of the year a company from Germany and that with our startup "culture"!?! Blickfeld GmbH and LiDAR
https://www.blickfeld.com/
Thank you, now I understand the award. I have read mechanics free LiDAR technology here already, but do not remember exactly where.Solid state LiDaR, no moving parts, electronically steered beam.
These days, "performance" is a 2 sided coin.Apologies if posted already
Redirecting
www-forbes-com.cdn.ampproject.org
View attachment 10587
View attachment 10590
Tata and Renesas strengthen their partnership
Party time, in the extreme, R577. Just where ever you are, please invite me over for a round or 2 ( hoping of course that your playing the short hand game, which I love ) and a serve of what's on offer, with some nice hot chillies, and a can or 3 of my favorite guinness, I'd be in heaven again, absolutely. The aces will surely appear for you often I reckon. Enjoy everything. Your living like a king IMHO.
Akida Ballista >>>>>> Royal Flush in the making, and 4oak already <<<<<<
hotty...
I’ve seen the term “Pantene” used often, but it’s meaning alludes me. Could someone please illuminate me?Pantene
I’ve seen the term “Pantene” used often, but it’s meaning alludes me. Could someone please illuminate me?
Maybe we need an acronyms/terminology page.
I am seriously surprised! Startup of the year a company from Germany and that with our startup "culture"!?! Blickfeld GmbH and LiDAR
https://www.blickfeld.com/
this articel is from 2020:Ja, aber im Jahr 2019 haben sie 19 Millionen Euro gesammelt, um sie auf ASIC zu implementieren.
![]()
France’s AnotherBrain raises €19 million Series A for their “OrganicAI”.
Deep learning company AnotherBrain has announced the first closing of their Series A, to the…tech.eu
OrganicAI™ wird derzeit als Software geliefert, aber das Unternehmen plant, die Technologie bald auf einem KI-Chip verfügbar zu machen.
Ihre Technologie sieht ziemlich gut aus, also sollten wir graben, um zu sehen, ob sie es geschafft haben, einen Chip zu produzieren.
Samsung Electronics
IP...![]()
Samsung kauft deutsche Firma – und wirft alle Mitarbeiter raus
Samsung Display hat die deutsche Firma Cynora übernommen, jetzt müssen alle der über 120 Mitarbeiter ihren Hut nehmen. Für Samsung ging..www.giga.de
This is German startup Culture
Love a drop of Guinness ... one for the pot and one for the ChefJust made a chocolate and wallnut brownie cake for deserts later as well, just needs popping in the oven and served with either custard or ice cream View attachment 10557
And one man size pot of stew that has been slow cooking for 5 hours and about to add the potatoes already for my poker playing friends later.
View attachment 10558
AWESOME find FF ... very nice ... love this bit! (I personally am thinking large Aerospace companies jumping on board) VERY EXCITINGWe have long been looking for official confirmation of a successful NASA Vorago Brainchip Phase 1 project.
Well I have found it and it is copied with a link below.
Note:
1. Vorago is Silicon Space Technology Corporation for newer shareholders.
2. Vorago used the term CNN RNN to describe AKIDA not SCNN.
As you read through the extracts you will note the following:
A. Vorago met all of the Phase 1 objectives
B. Vorago has five letters in support of continuing to the next Phase 2 importantly/interestingly two of these letters offer funding for the Phase 2 independent of NASA - (I personally am thinking large Aerospace companies jumping on board)
C. Vorago has modelling which shows AKIDA will allow NASA to have autonomous Rovers that will achieve speeds of up to 20 kph compared with a present speed of 4 centimetres a second.
There is in my opinion no other company on this planet with technology that can compete with the creation of Peter van der Made and Anil Mankar.
The Original Phase 1:
"The ultimate goal of this project is to create a radiation-hardened Neural Network suitable for Ede use. Neural Networks operating at the Edge will need to perform Continuous Learning and Few-shot/One-shot Learning with very low energy requirements, as will NN operation. Spiking Neural Networks (SNNs) provide the architectural framework to enable Edge operation and Continuous Learning. SNNs are event-driven and represent events as a spike or a train of spikes. Because of the sparsity of their data representation, the amount of processing Neural Networks need to do for the same stimulus can be significantly less than conventional Convolutional Neural Networks (CNNs), much like a human brain. To function in Space and in other extreme Edge environments, Neural Networks, including SNNs, must be made rad-hard.Brainchip’s Akida Event Domain Neural Processor (www.brainchipinc.com) offers native support for SNNs. Brainchip has been able to drive power consumption down to about 3 pJ per synaptic operation in their 28nm Si implementation. The Akida Development Environment (ADE) uses industry-standard development tools Tensorflow and Keras to allow easy simulation of its IP.Phase I is the first step towards creating radiation-hardened Edge AI capability. We plan to use the Akida Neural Processor architecture and, in Phase I, will: Understand the operation of Brainchip’s IP Understand 28nm instantiation of that IP (Akida) Evaluate radiation vulnerability of different parts of the IP through the Akida Development Environment Define architecture of target IC Define how HARDSIL® will be used to harden each chosen IP block Choose a target CMOS node (likely 28nm) and create a plan to design and fabricate the IC in that node, including defining the HARDSIL® process modules for this baseline process Define the radiation testing plan to establish the radiation robustness of the ICSuccessfully accomplishing these objectives:Establishes the feasibility of creating a useful, radiation-hardened product IC with embedded NPU and already-existing supporting software ecosystem to allow rapid adoption and productive use within NASA and the Space community.\n\n\n\n\t Creates the basis for an executable Phase II proposal and path towards fabrication of the processor."
CNN RNN Processor
FIRM: SILICON SPACE TECHNOLOGY CORPORATION PI: Jim Carlquist Proposal #:H6.22-4509
NON-PROPRIETARY DATA
Objectives:
The goal of this project is the creation of a radiation-hardened Spiking Neural Network (SNN) SoC based on the BrainChip Akida Neuron Fabric IP. Akida is a member of a small set of existing SNN architectures structured to more closely emulate computation in a human brain. The rationale for using a Spiking Neural Network (SNN) for Edge AI Computing is because of its efficiencies. The neurmorphic approach used in the Akida architecture takes fewer MACs per operation since it creates and uses sparsity of both weights and activation by its event-based model. In addition, Akida reduces memory consumption by quantizing and compressing network parameters. This also helps to reduce power consumption and die size while maintaining performance.
Spiking Neural Network Block Diagram
ACCOMPLISHMENTS
Notable Deliverables Provided:
• Design and Manufacturing Plans
• Radiation Testing Plan (included in Final report)
• Technical final report
Key Milestones Met
• Understand Akida Architecture
• Understand 28nm Implementation
• Evaluate Radiation Vulnerability of the IP Through the Akida
Development Environment
• Define Architecture of Target IC
• Define how HARDSIL® will be used in Target IC
• Create Design and Manufacturing Plans
• Define the Radiation Testing Plan to Establish the Radiation
Robustness of the IC
FUTURE PLANNED DEVELOPMENTS
Planned Post-Phase II Partners
We received five Letters of Support for this project.
Two of which will provide capital infusion to keep the project going, one for aid in radiation testing, and the final two for use in future space flights.
Planned/Possible Mission Infusion
NASA is keen to increase the performance of its autonomous rovers to allow for greater speeds.
Current routing methodologies limit speeds to 4cm/sec while NASA has a goal to be able to have autonomous rovers traverse at speeds up to 20km/hr.
Early calculations show the potential for this device to process several of the required neural network algorithms fast enough to meet this goal.
Planned/Possible Mission Commercialization
A detailed plan is included in the Phase I final submittal to commercialized a RADHARD flight ready QML, SNN SoC to be available for NASA and commercial use.
This plan will include a Phase II plus extensions to reach the commercialization goals we are seeking.
CONTRACT (CENTER): SUBTOPIC:
SOLICITATION-PHASE: TA:
80NSSC20C0365 (ARC)
H6.22 Deep Neural Net and Neuromorphic Processors for In- Space Autonomy and Cognition
SBIR 2020-I4.5.0 Autonomy
My opinion only DYOR
FF
AKIDA BALLISTA
We have long been looking for official confirmation of a successful NASA Vorago Brainchip Phase 1 project.
Well I have found it and it is copied with a link below.
Note:
1. Vorago is Silicon Space Technology Corporation for newer shareholders.
2. Vorago used the term CNN RNN to describe AKIDA not SCNN.
As you read through the extracts you will note the following:
A. Vorago met all of the Phase 1 objectives
B. Vorago has five letters in support of continuing to the next Phase 2 importantly/interestingly two of these letters offer funding for the Phase 2 independent of NASA - (I personally am thinking large Aerospace companies jumping on board)
C. Vorago has modelling which shows AKIDA will allow NASA to have autonomous Rovers that will achieve speeds of up to 20 kph compared with a present speed of 4 centimetres a second.
There is in my opinion no other company on this planet with technology that can compete with the creation of Peter van der Made and Anil Mankar.
The Original Phase 1:
"The ultimate goal of this project is to create a radiation-hardened Neural Network suitable for Ede use. Neural Networks operating at the Edge will need to perform Continuous Learning and Few-shot/One-shot Learning with very low energy requirements, as will NN operation. Spiking Neural Networks (SNNs) provide the architectural framework to enable Edge operation and Continuous Learning. SNNs are event-driven and represent events as a spike or a train of spikes. Because of the sparsity of their data representation, the amount of processing Neural Networks need to do for the same stimulus can be significantly less than conventional Convolutional Neural Networks (CNNs), much like a human brain. To function in Space and in other extreme Edge environments, Neural Networks, including SNNs, must be made rad-hard.Brainchip’s Akida Event Domain Neural Processor (www.brainchipinc.com) offers native support for SNNs. Brainchip has been able to drive power consumption down to about 3 pJ per synaptic operation in their 28nm Si implementation. The Akida Development Environment (ADE) uses industry-standard development tools Tensorflow and Keras to allow easy simulation of its IP.Phase I is the first step towards creating radiation-hardened Edge AI capability. We plan to use the Akida Neural Processor architecture and, in Phase I, will: Understand the operation of Brainchip’s IP Understand 28nm instantiation of that IP (Akida) Evaluate radiation vulnerability of different parts of the IP through the Akida Development Environment Define architecture of target IC Define how HARDSIL® will be used to harden each chosen IP block Choose a target CMOS node (likely 28nm) and create a plan to design and fabricate the IC in that node, including defining the HARDSIL® process modules for this baseline process Define the radiation testing plan to establish the radiation robustness of the ICSuccessfully accomplishing these objectives:Establishes the feasibility of creating a useful, radiation-hardened product IC with embedded NPU and already-existing supporting software ecosystem to allow rapid adoption and productive use within NASA and the Space community.\n\n\n\n\t Creates the basis for an executable Phase II proposal and path towards fabrication of the processor."
CNN RNN Processor
FIRM: SILICON SPACE TECHNOLOGY CORPORATION PI: Jim Carlquist Proposal #:H6.22-4509
NON-PROPRIETARY DATA
Objectives:
The goal of this project is the creation of a radiation-hardened Spiking Neural Network (SNN) SoC based on the BrainChip Akida Neuron Fabric IP. Akida is a member of a small set of existing SNN architectures structured to more closely emulate computation in a human brain. The rationale for using a Spiking Neural Network (SNN) for Edge AI Computing is because of its efficiencies. The neurmorphic approach used in the Akida architecture takes fewer MACs per operation since it creates and uses sparsity of both weights and activation by its event-based model. In addition, Akida reduces memory consumption by quantizing and compressing network parameters. This also helps to reduce power consumption and die size while maintaining performance.
Spiking Neural Network Block Diagram
ACCOMPLISHMENTS
Notable Deliverables Provided:
• Design and Manufacturing Plans
• Radiation Testing Plan (included in Final report)
• Technical final report
Key Milestones Met
• Understand Akida Architecture
• Understand 28nm Implementation
• Evaluate Radiation Vulnerability of the IP Through the Akida
Development Environment
• Define Architecture of Target IC
• Define how HARDSIL® will be used in Target IC
• Create Design and Manufacturing Plans
• Define the Radiation Testing Plan to Establish the Radiation
Robustness of the IC
FUTURE PLANNED DEVELOPMENTS
Planned Post-Phase II Partners
We received five Letters of Support for this project.
Two of which will provide capital infusion to keep the project going, one for aid in radiation testing, and the final two for use in future space flights.
Planned/Possible Mission Infusion
NASA is keen to increase the performance of its autonomous rovers to allow for greater speeds.
Current routing methodologies limit speeds to 4cm/sec while NASA has a goal to be able to have autonomous rovers traverse at speeds up to 20km/hr.
Early calculations show the potential for this device to process several of the required neural network algorithms fast enough to meet this goal.
Planned/Possible Mission Commercialization
A detailed plan is included in the Phase I final submittal to commercialized a RADHARD flight ready QML, SNN SoC to be available for NASA and commercial use.
This plan will include a Phase II plus extensions to reach the commercialization goals we are seeking.
CONTRACT (CENTER): SUBTOPIC:
SOLICITATION-PHASE: TA:
80NSSC20C0365 (ARC)
H6.22 Deep Neural Net and Neuromorphic Processors for In- Space Autonomy and Cognition
SBIR 2020-I4.5.0 Autonomy
My opinion only DYOR
FF
Wow to you @Fact Finder and to NASA with a goal of its rovers to do 20km per hour. They will definitely need Akida to achieve this self driving.We have long been looking for official confirmation of a successful NASA Vorago Brainchip Phase 1 project.
Well I have found it and it is copied with a link below.
Note:
1. Vorago is Silicon Space Technology Corporation for newer shareholders.
2. Vorago used the term CNN RNN to describe AKIDA not SCNN.
As you read through the extracts you will note the following:
A. Vorago met all of the Phase 1 objectives
B. Vorago has five letters in support of continuing to the next Phase 2 importantly/interestingly two of these letters offer funding for the Phase 2 independent of NASA - (I personally am thinking large Aerospace companies jumping on board)
C. Vorago has modelling which shows AKIDA will allow NASA to have autonomous Rovers that will achieve speeds of up to 20 kph compared with a present speed of 4 centimetres a second.
There is in my opinion no other company on this planet with technology that can compete with the creation of Peter van der Made and Anil Mankar.
The Original Phase 1:
"The ultimate goal of this project is to create a radiation-hardened Neural Network suitable for Ede use. Neural Networks operating at the Edge will need to perform Continuous Learning and Few-shot/One-shot Learning with very low energy requirements, as will NN operation. Spiking Neural Networks (SNNs) provide the architectural framework to enable Edge operation and Continuous Learning. SNNs are event-driven and represent events as a spike or a train of spikes. Because of the sparsity of their data representation, the amount of processing Neural Networks need to do for the same stimulus can be significantly less than conventional Convolutional Neural Networks (CNNs), much like a human brain. To function in Space and in other extreme Edge environments, Neural Networks, including SNNs, must be made rad-hard.Brainchip’s Akida Event Domain Neural Processor (www.brainchipinc.com) offers native support for SNNs. Brainchip has been able to drive power consumption down to about 3 pJ per synaptic operation in their 28nm Si implementation. The Akida Development Environment (ADE) uses industry-standard development tools Tensorflow and Keras to allow easy simulation of its IP.Phase I is the first step towards creating radiation-hardened Edge AI capability. We plan to use the Akida Neural Processor architecture and, in Phase I, will: Understand the operation of Brainchip’s IP Understand 28nm instantiation of that IP (Akida) Evaluate radiation vulnerability of different parts of the IP through the Akida Development Environment Define architecture of target IC Define how HARDSIL® will be used to harden each chosen IP block Choose a target CMOS node (likely 28nm) and create a plan to design and fabricate the IC in that node, including defining the HARDSIL® process modules for this baseline process Define the radiation testing plan to establish the radiation robustness of the ICSuccessfully accomplishing these objectives:Establishes the feasibility of creating a useful, radiation-hardened product IC with embedded NPU and already-existing supporting software ecosystem to allow rapid adoption and productive use within NASA and the Space community.\n\n\n\n\t Creates the basis for an executable Phase II proposal and path towards fabrication of the processor."
CNN RNN Processor
FIRM: SILICON SPACE TECHNOLOGY CORPORATION PI: Jim Carlquist Proposal #:H6.22-4509
NON-PROPRIETARY DATA
Objectives:
The goal of this project is the creation of a radiation-hardened Spiking Neural Network (SNN) SoC based on the BrainChip Akida Neuron Fabric IP. Akida is a member of a small set of existing SNN architectures structured to more closely emulate computation in a human brain. The rationale for using a Spiking Neural Network (SNN) for Edge AI Computing is because of its efficiencies. The neurmorphic approach used in the Akida architecture takes fewer MACs per operation since it creates and uses sparsity of both weights and activation by its event-based model. In addition, Akida reduces memory consumption by quantizing and compressing network parameters. This also helps to reduce power consumption and die size while maintaining performance.
Spiking Neural Network Block Diagram
ACCOMPLISHMENTS
Notable Deliverables Provided:
• Design and Manufacturing Plans
• Radiation Testing Plan (included in Final report)
• Technical final report
Key Milestones Met
• Understand Akida Architecture
• Understand 28nm Implementation
• Evaluate Radiation Vulnerability of the IP Through the Akida
Development Environment
• Define Architecture of Target IC
• Define how HARDSIL® will be used in Target IC
• Create Design and Manufacturing Plans
• Define the Radiation Testing Plan to Establish the Radiation
Robustness of the IC
FUTURE PLANNED DEVELOPMENTS
Planned Post-Phase II Partners
We received five Letters of Support for this project.
Two of which will provide capital infusion to keep the project going, one for aid in radiation testing, and the final two for use in future space flights.
Planned/Possible Mission Infusion
NASA is keen to increase the performance of its autonomous rovers to allow for greater speeds.
Current routing methodologies limit speeds to 4cm/sec while NASA has a goal to be able to have autonomous rovers traverse at speeds up to 20km/hr.
Early calculations show the potential for this device to process several of the required neural network algorithms fast enough to meet this goal.
Planned/Possible Mission Commercialization
A detailed plan is included in the Phase I final submittal to commercialized a RADHARD flight ready QML, SNN SoC to be available for NASA and commercial use.
This plan will include a Phase II plus extensions to reach the commercialization goals we are seeking.
CONTRACT (CENTER): SUBTOPIC:
SOLICITATION-PHASE: TA:
80NSSC20C0365 (ARC)
H6.22 Deep Neural Net and Neuromorphic Processors for In- Space Autonomy and Cognition
SBIR 2020-I4.5.0 Autonomy
My opinion only DYOR
FF
AKIDA BALLISTA
My mind immediately thinks who else has read this nugget of a find. Nice work FF.We have long been looking for official confirmation of a successful NASA Vorago Brainchip Phase 1 project.
Well I have found it and it is copied with a link below.
Note:
1. Vorago is Silicon Space Technology Corporation for newer shareholders.
2. Vorago used the term CNN RNN to describe AKIDA not SCNN.
As you read through the extracts you will note the following:
A. Vorago met all of the Phase 1 objectives
B. Vorago has five letters in support of continuing to the next Phase 2 importantly/interestingly two of these letters offer funding for the Phase 2 independent of NASA - (I personally am thinking large Aerospace companies jumping on board)
C. Vorago has modelling which shows AKIDA will allow NASA to have autonomous Rovers that will achieve speeds of up to 20 kph compared with a present speed of 4 centimetres a second.
There is in my opinion no other company on this planet with technology that can compete with the creation of Peter van der Made and Anil Mankar.
The Original Phase 1:
"The ultimate goal of this project is to create a radiation-hardened Neural Network suitable for Ede use. Neural Networks operating at the Edge will need to perform Continuous Learning and Few-shot/One-shot Learning with very low energy requirements, as will NN operation. Spiking Neural Networks (SNNs) provide the architectural framework to enable Edge operation and Continuous Learning. SNNs are event-driven and represent events as a spike or a train of spikes. Because of the sparsity of their data representation, the amount of processing Neural Networks need to do for the same stimulus can be significantly less than conventional Convolutional Neural Networks (CNNs), much like a human brain. To function in Space and in other extreme Edge environments, Neural Networks, including SNNs, must be made rad-hard.Brainchip’s Akida Event Domain Neural Processor (www.brainchipinc.com) offers native support for SNNs. Brainchip has been able to drive power consumption down to about 3 pJ per synaptic operation in their 28nm Si implementation. The Akida Development Environment (ADE) uses industry-standard development tools Tensorflow and Keras to allow easy simulation of its IP.Phase I is the first step towards creating radiation-hardened Edge AI capability. We plan to use the Akida Neural Processor architecture and, in Phase I, will: Understand the operation of Brainchip’s IP Understand 28nm instantiation of that IP (Akida) Evaluate radiation vulnerability of different parts of the IP through the Akida Development Environment Define architecture of target IC Define how HARDSIL® will be used to harden each chosen IP block Choose a target CMOS node (likely 28nm) and create a plan to design and fabricate the IC in that node, including defining the HARDSIL® process modules for this baseline process Define the radiation testing plan to establish the radiation robustness of the ICSuccessfully accomplishing these objectives:Establishes the feasibility of creating a useful, radiation-hardened product IC with embedded NPU and already-existing supporting software ecosystem to allow rapid adoption and productive use within NASA and the Space community.\n\n\n\n\t Creates the basis for an executable Phase II proposal and path towards fabrication of the processor."
CNN RNN Processor
FIRM: SILICON SPACE TECHNOLOGY CORPORATION PI: Jim Carlquist Proposal #:H6.22-4509
NON-PROPRIETARY DATA
Objectives:
The goal of this project is the creation of a radiation-hardened Spiking Neural Network (SNN) SoC based on the BrainChip Akida Neuron Fabric IP. Akida is a member of a small set of existing SNN architectures structured to more closely emulate computation in a human brain. The rationale for using a Spiking Neural Network (SNN) for Edge AI Computing is because of its efficiencies. The neurmorphic approach used in the Akida architecture takes fewer MACs per operation since it creates and uses sparsity of both weights and activation by its event-based model. In addition, Akida reduces memory consumption by quantizing and compressing network parameters. This also helps to reduce power consumption and die size while maintaining performance.
Spiking Neural Network Block Diagram
ACCOMPLISHMENTS
Notable Deliverables Provided:
• Design and Manufacturing Plans
• Radiation Testing Plan (included in Final report)
• Technical final report
Key Milestones Met
• Understand Akida Architecture
• Understand 28nm Implementation
• Evaluate Radiation Vulnerability of the IP Through the Akida
Development Environment
• Define Architecture of Target IC
• Define how HARDSIL® will be used in Target IC
• Create Design and Manufacturing Plans
• Define the Radiation Testing Plan to Establish the Radiation
Robustness of the IC
FUTURE PLANNED DEVELOPMENTS
Planned Post-Phase II Partners
We received five Letters of Support for this project.
Two of which will provide capital infusion to keep the project going, one for aid in radiation testing, and the final two for use in future space flights.
Planned/Possible Mission Infusion
NASA is keen to increase the performance of its autonomous rovers to allow for greater speeds.
Current routing methodologies limit speeds to 4cm/sec while NASA has a goal to be able to have autonomous rovers traverse at speeds up to 20km/hr.
Early calculations show the potential for this device to process several of the required neural network algorithms fast enough to meet this goal.
Planned/Possible Mission Commercialization
A detailed plan is included in the Phase I final submittal to commercialized a RADHARD flight ready QML, SNN SoC to be available for NASA and commercial use.
This plan will include a Phase II plus extensions to reach the commercialization goals we are seeking.
CONTRACT (CENTER): SUBTOPIC:
SOLICITATION-PHASE: TA:
80NSSC20C0365 (ARC)
H6.22 Deep Neural Net and Neuromorphic Processors for In- Space Autonomy and Cognition
SBIR 2020-I4.5.0 Autonomy
My opinion only DYOR
FF
AKIDA BALLISTA
Facts found by the Finder. Great stuff FF. Don’t doubt the technology people. We got 12 winning months ahead of us this financial year.We have long been looking for official confirmation of a successful NASA Vorago Brainchip Phase 1 project.
Well I have found it and it is copied with a link below.
Note:
1. Vorago is Silicon Space Technology Corporation for newer shareholders.
2. Vorago used the term CNN RNN to describe AKIDA not SCNN.
As you read through the extracts you will note the following:
A. Vorago met all of the Phase 1 objectives
B. Vorago has five letters in support of continuing to the next Phase 2 importantly/interestingly two of these letters offer funding for the Phase 2 independent of NASA - (I personally am thinking large Aerospace companies jumping on board)
C. Vorago has modelling which shows AKIDA will allow NASA to have autonomous Rovers that will achieve speeds of up to 20 kph compared with a present speed of 4 centimetres a second.
There is in my opinion no other company on this planet with technology that can compete with the creation of Peter van der Made and Anil Mankar.
The Original Phase 1:
"The ultimate goal of this project is to create a radiation-hardened Neural Network suitable for Ede use. Neural Networks operating at the Edge will need to perform Continuous Learning and Few-shot/One-shot Learning with very low energy requirements, as will NN operation. Spiking Neural Networks (SNNs) provide the architectural framework to enable Edge operation and Continuous Learning. SNNs are event-driven and represent events as a spike or a train of spikes. Because of the sparsity of their data representation, the amount of processing Neural Networks need to do for the same stimulus can be significantly less than conventional Convolutional Neural Networks (CNNs), much like a human brain. To function in Space and in other extreme Edge environments, Neural Networks, including SNNs, must be made rad-hard.Brainchip’s Akida Event Domain Neural Processor (www.brainchipinc.com) offers native support for SNNs. Brainchip has been able to drive power consumption down to about 3 pJ per synaptic operation in their 28nm Si implementation. The Akida Development Environment (ADE) uses industry-standard development tools Tensorflow and Keras to allow easy simulation of its IP.Phase I is the first step towards creating radiation-hardened Edge AI capability. We plan to use the Akida Neural Processor architecture and, in Phase I, will: Understand the operation of Brainchip’s IP Understand 28nm instantiation of that IP (Akida) Evaluate radiation vulnerability of different parts of the IP through the Akida Development Environment Define architecture of target IC Define how HARDSIL® will be used to harden each chosen IP block Choose a target CMOS node (likely 28nm) and create a plan to design and fabricate the IC in that node, including defining the HARDSIL® process modules for this baseline process Define the radiation testing plan to establish the radiation robustness of the ICSuccessfully accomplishing these objectives:Establishes the feasibility of creating a useful, radiation-hardened product IC with embedded NPU and already-existing supporting software ecosystem to allow rapid adoption and productive use within NASA and the Space community.\n\n\n\n\t Creates the basis for an executable Phase II proposal and path towards fabrication of the processor."
CNN RNN Processor
FIRM: SILICON SPACE TECHNOLOGY CORPORATION PI: Jim Carlquist Proposal #:H6.22-4509
NON-PROPRIETARY DATA
Objectives:
The goal of this project is the creation of a radiation-hardened Spiking Neural Network (SNN) SoC based on the BrainChip Akida Neuron Fabric IP. Akida is a member of a small set of existing SNN architectures structured to more closely emulate computation in a human brain. The rationale for using a Spiking Neural Network (SNN) for Edge AI Computing is because of its efficiencies. The neurmorphic approach used in the Akida architecture takes fewer MACs per operation since it creates and uses sparsity of both weights and activation by its event-based model. In addition, Akida reduces memory consumption by quantizing and compressing network parameters. This also helps to reduce power consumption and die size while maintaining performance.
Spiking Neural Network Block Diagram
ACCOMPLISHMENTS
Notable Deliverables Provided:
• Design and Manufacturing Plans
• Radiation Testing Plan (included in Final report)
• Technical final report
Key Milestones Met
• Understand Akida Architecture
• Understand 28nm Implementation
• Evaluate Radiation Vulnerability of the IP Through the Akida
Development Environment
• Define Architecture of Target IC
• Define how HARDSIL® will be used in Target IC
• Create Design and Manufacturing Plans
• Define the Radiation Testing Plan to Establish the Radiation
Robustness of the IC
FUTURE PLANNED DEVELOPMENTS
Planned Post-Phase II Partners
We received five Letters of Support for this project.
Two of which will provide capital infusion to keep the project going, one for aid in radiation testing, and the final two for use in future space flights.
Planned/Possible Mission Infusion
NASA is keen to increase the performance of its autonomous rovers to allow for greater speeds.
Current routing methodologies limit speeds to 4cm/sec while NASA has a goal to be able to have autonomous rovers traverse at speeds up to 20km/hr.
Early calculations show the potential for this device to process several of the required neural network algorithms fast enough to meet this goal.
Planned/Possible Mission Commercialization
A detailed plan is included in the Phase I final submittal to commercialized a RADHARD flight ready QML, SNN SoC to be available for NASA and commercial use.
This plan will include a Phase II plus extensions to reach the commercialization goals we are seeking.
CONTRACT (CENTER): SUBTOPIC:
SOLICITATION-PHASE: TA:
80NSSC20C0365 (ARC)
H6.22 Deep Neural Net and Neuromorphic Processors for In- Space Autonomy and Cognition
SBIR 2020-I4.5.0 Autonomy
My opinion only DYOR
FF
AKIDA BALLISTA