Bravo
If ARM was an arm, BRN would be its biceps💪!
Humans Are Sending Their First AI in Space, You (Probably) Have Nothing to Worry About
Published: 12 Apr 2024, 06:52 UTC• By:Daniel Patrascu

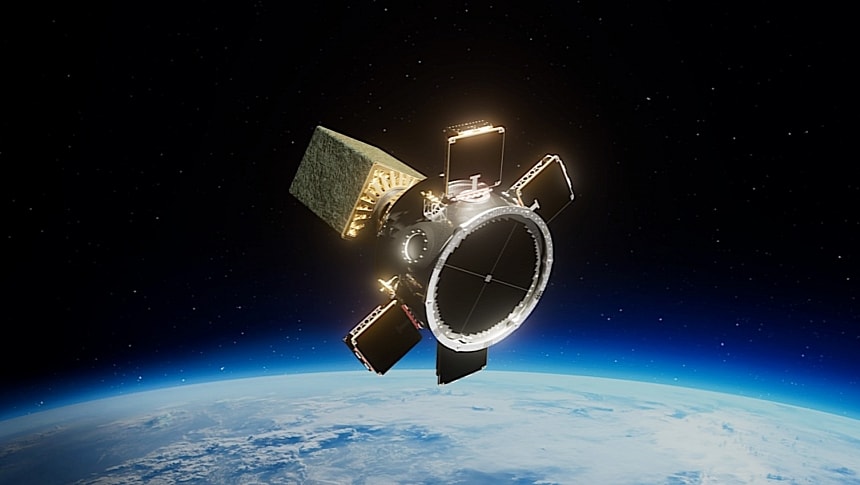
Photo: Firefly
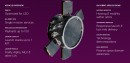
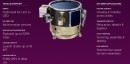
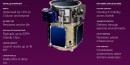
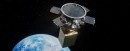
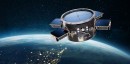
Artificial intelligence with various degrees of smarts are pretty much in everything these days. From text generators to the systems that power the cameras in your phone, AIs are slowly becoming humanity's most trusted sidekicks. To our knowledge, though, a full-fledged AI has never traveled to space. That will change as soon as this year.
There's a good chance the name Klepsydra Technologies doesn't ring that many bells. It's a company that describes itself as the maker of high-performance software for edge computing. And one of its products is an AI.
The tech is marketed as a high-performance deep neural network engine meant to drive edge computers in a stable, low latency, are relatively affordable manner. It can be used for robotic vision-based navigation, 3D model analysis of infrastructures, surveillance, and data quality checks.
It is this Klepsydra that will become humanity's first AI to be deployed in space. That's because it will fly later this year on board a spacecraft called Elytra.
Elytra is more or less a family of space trucks (or SUVs, because its official name is Space Utility Vehicle). Manufactured by Firefly Aerospace, it is part of a new generation of space hardware meant to provide in-orbit logistics support. This means stuff like satellite servicing and refueling, among other things.
There are three Elytra variants, namely the Dawn, Dusk, and Dark, each with their own capabilities in terms of the orbits they can reach and the tasks they are capable of accomplishing.
The Dawn, for instance, is meant for use in low-Earth orbit as a tool for delivery missions, while Dusk can reach geosynchronous orbit to relocate satellites or de-orbit them. Last but not least, Dark has been designed to assist orbital infrastructure and act as a tug for hardware transfer from low-Earth orbit to lunar orbit.
Later this year one of the Elytra SUVs will get to fly for the first time. Launched on top of a rocket called Alpha (also made by Firefly), it will be hosting the Klepsydra AI. The goal of the mission is to test the computer software's ability to navigate.
As per the details released by the two companies, the Klepsydra will be used to process sensor data onboard and relay the processed data back to Earth. The data being worked pertains to real-time attitude determination, guidance, navigation, and control of the spacecraft.
Separately, the AI will also be testing its ability to "dynamically switch AI applications onboard," but the specifics of this mission were not disclosed.
The AI will spend up to a year in Earth orbit, acting as "an edge computing platform for hosted software customers."It will be accompanied on board the Elytra by a series of other payloads, including some being sent up by the U.S. government.
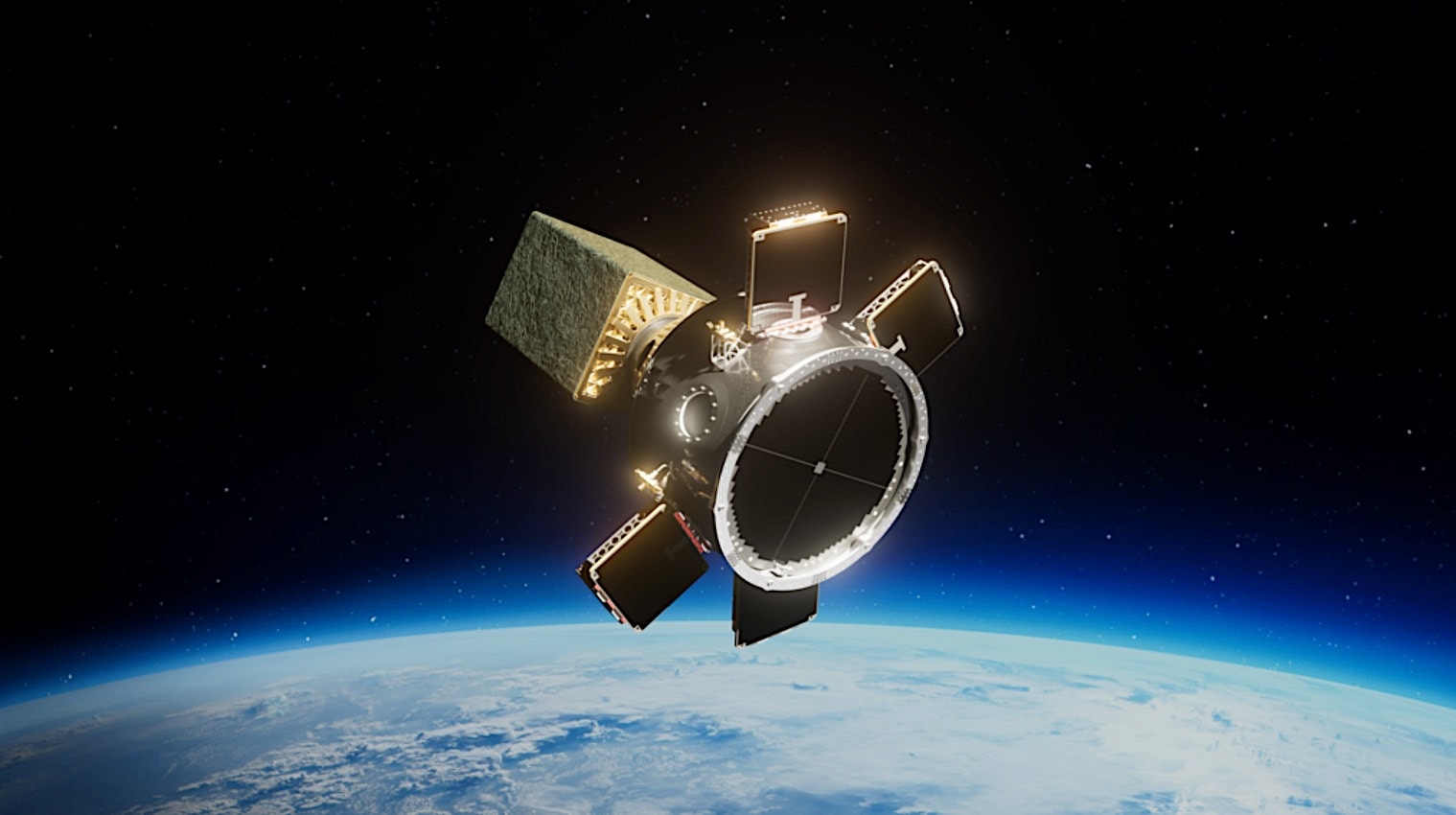
Humans Are Sending Their First AI in Space, You (Probably) Have Nothing to Worry About
The first Firefly Aerospace Elytra mission scheduled for later this year will carry with it an artificial intelligence developed by a company called Klepsydra
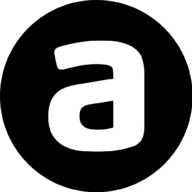