An old interview, but good snapshot summary of CNN vs SNN (GPU v Akida) and fintech applications…
Anna Reitman
FacebookTwitterLinkedInEmail
The application of artificial intelligence across industries depends on hardware acceleration, and some of the ways that’s being done was detailed at a recent Nvidia conference for GPU (graphics processing unit) developers, held in Tel Aviv.
But the advance of neural networks is itself branching out, from the computationally intensive convoluted neural networks (CNNs) to the emerging spiking neural networks (SNNs), also known as neuromorphic computing.
Nvidia’s chief scientist, Bill Dally, was dismissive of the challenge presented by SNNs, saying that because the design is inspired by biological systems, computation is analog, and therefore inefficient. He compared it to “making airplanes that flap wings.”
Robert Beachler, SVP, BrainChip
ASX-listed firm BrainChip recently announced that it’s going to market with the Akida Neuromorphic System-on-Chip (NSoC) for embedded vision, cybersecurity, and fintech, targeting a $10 price point for the chip.
We asked Robert Beachler, SVP of marketing and business development to explain how SNNs are developing for fintech applications.
Fintech Capital Markets: How would you respond to Bill Dally’s description of SNNs?
Robert Beachler: His thesis is that SNNs need to use analog computational elements to be efficient. This is an incorrect statement. BrainChip’s neuron model is purely digital, and BrainChip accelerates spiking neural networks in a digital manner.
On representing numbers as spikes: This is a common misperception that spokespeople working with CNNs have of SNNs. In CNNs they use numbers to represent data and therefore think that SNNs need to use numbers as well – which is incorrect.
Neurons don’t think in mathematical and floating point values, which is why humans invented calculators and computers – we recognized that the human mind is not good at complex math.
BrainChip’s data-to-spike converters take the external information (whether it is pixels, audio, fintech data, etc.) and translates it to a series of spikes. This translation does not encode the values of numbers.
Rather, the spike series represent pertinent information such as frequency, magnitude change, or other characteristics. This is what sensory organs do, like the retina, cochlea, etc.; they don’t send numbers – they send spike series that represent the pertinent information.
Source: BrainChip
The reason CNNs take so much power and silicon real estate is that they try to emulate what neurons perform using complex math functions – taking hundreds of watts. SNNs, by contrast, are more efficient as they more closely emulate the most efficient thinking machine – the human mind, which only uses 20 watts.
And regarding planes that flap and have feathers: This is a rather elementary attack that shows a lack of understanding of how state-of-the-art SNNs operate. If you tried to exactly model the complex bio-electrical process of the human neuron, like electron potentials, ion channels, dopamine reactions, etc., you would indeed have a very complex and inefficient neuron model.
But if you emulate only the most important neuron characteristics, synaptic connections and firing thresholds, in a purely digital manner, you end up with a very efficient neuron model, which BrainChip has been researching for many years. That is why the BrainChip neuromorphic system-on-chip is an order of magnitude more efficient on an images / second / watt basis compared to GPUs and FPGAs (field-programmable gate arrays).
FTCM: Can you describe the difference between CNNs and SNNs?
RB: Traditional computer architecture is really set up for sequential execution, whereas an artificial neural network is a distributed parallel feed-forward architecture that doesn’t lend itself to sequential execution; it really wants to be distributed, it wants to be a lot of compute in parallel. And the primary difference between a CNN and an SNN is what is being done in the neuron itself.
With CNNs, it’s essentially a filtering operation. At its base, it is matrix multiplication, so all of the CNN acceleration chips are really just looking at ways to do more multiply accumulates with lower amounts of silicon and lower amounts of power consumption.
For SNNs, instead of using this math function, the actual neuron that we use is modeled after biology where you have synapses and neurons, and the data between the synapses are spikes in the brain. The way that it learns is that you reinforce or inhibit these synaptic connections and then you also can change the firing threshold of the neuron itself. The chip learns through reinforcement and inhibition of synaptic connections and the thresholds.
Source: BrainChip
FTCM: Can you give me an example that makes sense for finance?
RB: In the case of financial technology, there’s a lot of unlabeled data. What these neural networks will do is cluster things that are similar together, and that could be successful trading patterns, economic indicators, it could be basically just about any type of data that financial traders or economists want to look at.
There’s an entire science of converting data into spikes. We have embedded in our chips certain data-to-spike conversions for specific applications, but for fintech it’s more of a general data-to-spike conversion, that takes generic data and puts it into a time series of spikes. Inside, on a neuron fabric, a spiking neural network model is doing pattern recognition in an unsupervised mode using unlabeled data.
FTCM: One of the first things I think of is the “black box” problem. Can you see how decisions get made?
RB: That’s really a conversation that hasn’t been solved, because it’s really hard to understand; you have these deep neural networks, multiple layers, looking inside of them to see what it’s doing. It’s very hard to visualize and it’s not something that you really can do very well.
It’s really a self-organizing, self-training type of system, and you’re right, it’s very difficult to explain the pattern.
FTCM: This is commercially available now, right? Can you tell us about your financial services clients?
RB: We have the development environment that’s available now for our customers to start creating the SNNs; we anticipate sampling the silicon mid-2019. We are focusing on three areas: vision systems, cybersecurity and fintech.
The financial sector is a rarefied environment; it’s not going to be thousands of customers, whereas there can be thousands of drones and vision-guided robotic systems. Our fintech and cybersecurity will be a smaller percentage in terms of number of customers, but it might be higher in terms of the potential revenue size for us because they would be buying lots of chips in big groups for servers. I can’t say anything more specific at the moment.
In the fintech community, there’s not a lot of public domain information about what are the neural networks that these companies are using. They are in the business of making money and they are really protecting that intellectual property.
FTCM: And what does it actually look like to set up?
RB: In most fintech applications, it would be a PCI Express card that may have between 20 and 50 of these chips in order to be able to execute very large neural networks very quickly.
We measure things in terms of neurons, and the neuron fabric has 1.2 million neurons and 10 billion synapses. It has a lot of compute capability. When we talk about replacing math-intensive convolutions of backpropagation methods, we are talking about replacing those matrices with the neurons and the synapses.
Each neuron-processing core can do a certain number of neurons and synapses. We made their functionality to accelerate this computation but also to be able to emulate a lot of the convolutional neural network styles.
Source: BrainChip
FTCM: How do you see the future unfolding for the different kinds of hardware acceleration architecture?
RB: You’re seeing a melding of the different technologies on any given chip. If you were to look into the future, you could have an ARM processor (architecture for systems-on-chips) and an embedded FPGA for data manipulation and scaling, then you have a neuron fabric which is either accelerating SNNs and CNNs, and that all comes together in a monolithic piece of silicon.
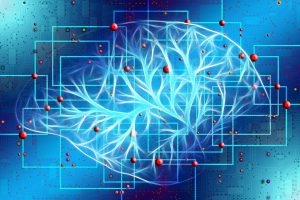
AI tech: are your neural networks convoluted? Try spikes.
October 26, 2018Anna Reitman
FacebookTwitterLinkedInEmail
The application of artificial intelligence across industries depends on hardware acceleration, and some of the ways that’s being done was detailed at a recent Nvidia conference for GPU (graphics processing unit) developers, held in Tel Aviv.
But the advance of neural networks is itself branching out, from the computationally intensive convoluted neural networks (CNNs) to the emerging spiking neural networks (SNNs), also known as neuromorphic computing.
Nvidia’s chief scientist, Bill Dally, was dismissive of the challenge presented by SNNs, saying that because the design is inspired by biological systems, computation is analog, and therefore inefficient. He compared it to “making airplanes that flap wings.”
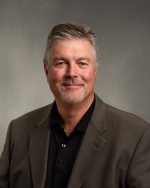
Robert Beachler, SVP, BrainChip
ASX-listed firm BrainChip recently announced that it’s going to market with the Akida Neuromorphic System-on-Chip (NSoC) for embedded vision, cybersecurity, and fintech, targeting a $10 price point for the chip.
We asked Robert Beachler, SVP of marketing and business development to explain how SNNs are developing for fintech applications.
Fintech Capital Markets: How would you respond to Bill Dally’s description of SNNs?
Robert Beachler: His thesis is that SNNs need to use analog computational elements to be efficient. This is an incorrect statement. BrainChip’s neuron model is purely digital, and BrainChip accelerates spiking neural networks in a digital manner.
On representing numbers as spikes: This is a common misperception that spokespeople working with CNNs have of SNNs. In CNNs they use numbers to represent data and therefore think that SNNs need to use numbers as well – which is incorrect.
Neurons don’t think in mathematical and floating point values, which is why humans invented calculators and computers – we recognized that the human mind is not good at complex math.
BrainChip’s data-to-spike converters take the external information (whether it is pixels, audio, fintech data, etc.) and translates it to a series of spikes. This translation does not encode the values of numbers.
Rather, the spike series represent pertinent information such as frequency, magnitude change, or other characteristics. This is what sensory organs do, like the retina, cochlea, etc.; they don’t send numbers – they send spike series that represent the pertinent information.
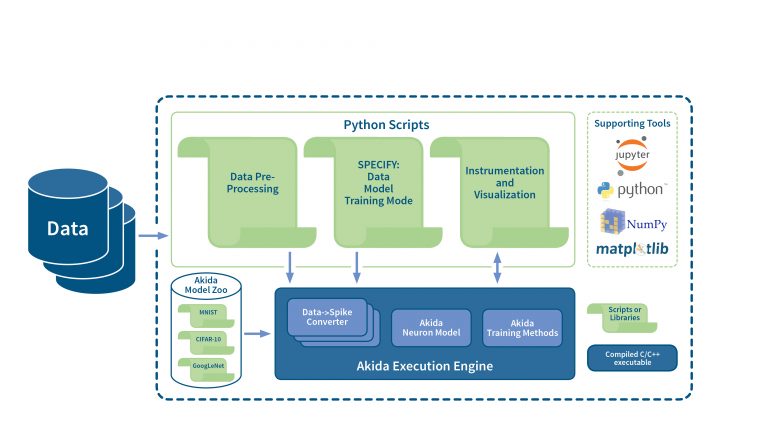
Source: BrainChip
The reason CNNs take so much power and silicon real estate is that they try to emulate what neurons perform using complex math functions – taking hundreds of watts. SNNs, by contrast, are more efficient as they more closely emulate the most efficient thinking machine – the human mind, which only uses 20 watts.
And regarding planes that flap and have feathers: This is a rather elementary attack that shows a lack of understanding of how state-of-the-art SNNs operate. If you tried to exactly model the complex bio-electrical process of the human neuron, like electron potentials, ion channels, dopamine reactions, etc., you would indeed have a very complex and inefficient neuron model.
But if you emulate only the most important neuron characteristics, synaptic connections and firing thresholds, in a purely digital manner, you end up with a very efficient neuron model, which BrainChip has been researching for many years. That is why the BrainChip neuromorphic system-on-chip is an order of magnitude more efficient on an images / second / watt basis compared to GPUs and FPGAs (field-programmable gate arrays).
FTCM: Can you describe the difference between CNNs and SNNs?
RB: Traditional computer architecture is really set up for sequential execution, whereas an artificial neural network is a distributed parallel feed-forward architecture that doesn’t lend itself to sequential execution; it really wants to be distributed, it wants to be a lot of compute in parallel. And the primary difference between a CNN and an SNN is what is being done in the neuron itself.
With CNNs, it’s essentially a filtering operation. At its base, it is matrix multiplication, so all of the CNN acceleration chips are really just looking at ways to do more multiply accumulates with lower amounts of silicon and lower amounts of power consumption.
For SNNs, instead of using this math function, the actual neuron that we use is modeled after biology where you have synapses and neurons, and the data between the synapses are spikes in the brain. The way that it learns is that you reinforce or inhibit these synaptic connections and then you also can change the firing threshold of the neuron itself. The chip learns through reinforcement and inhibition of synaptic connections and the thresholds.
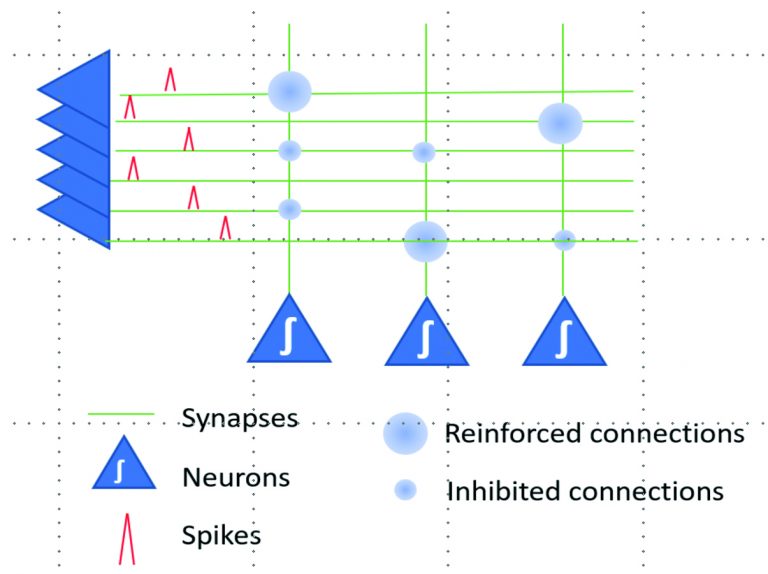
Source: BrainChip
FTCM: Can you give me an example that makes sense for finance?
RB: In the case of financial technology, there’s a lot of unlabeled data. What these neural networks will do is cluster things that are similar together, and that could be successful trading patterns, economic indicators, it could be basically just about any type of data that financial traders or economists want to look at.
There’s an entire science of converting data into spikes. We have embedded in our chips certain data-to-spike conversions for specific applications, but for fintech it’s more of a general data-to-spike conversion, that takes generic data and puts it into a time series of spikes. Inside, on a neuron fabric, a spiking neural network model is doing pattern recognition in an unsupervised mode using unlabeled data.
FTCM: One of the first things I think of is the “black box” problem. Can you see how decisions get made?
RB: That’s really a conversation that hasn’t been solved, because it’s really hard to understand; you have these deep neural networks, multiple layers, looking inside of them to see what it’s doing. It’s very hard to visualize and it’s not something that you really can do very well.
It’s really a self-organizing, self-training type of system, and you’re right, it’s very difficult to explain the pattern.
FTCM: This is commercially available now, right? Can you tell us about your financial services clients?
RB: We have the development environment that’s available now for our customers to start creating the SNNs; we anticipate sampling the silicon mid-2019. We are focusing on three areas: vision systems, cybersecurity and fintech.
The financial sector is a rarefied environment; it’s not going to be thousands of customers, whereas there can be thousands of drones and vision-guided robotic systems. Our fintech and cybersecurity will be a smaller percentage in terms of number of customers, but it might be higher in terms of the potential revenue size for us because they would be buying lots of chips in big groups for servers. I can’t say anything more specific at the moment.
In the fintech community, there’s not a lot of public domain information about what are the neural networks that these companies are using. They are in the business of making money and they are really protecting that intellectual property.
FTCM: And what does it actually look like to set up?
RB: In most fintech applications, it would be a PCI Express card that may have between 20 and 50 of these chips in order to be able to execute very large neural networks very quickly.
We measure things in terms of neurons, and the neuron fabric has 1.2 million neurons and 10 billion synapses. It has a lot of compute capability. When we talk about replacing math-intensive convolutions of backpropagation methods, we are talking about replacing those matrices with the neurons and the synapses.
Each neuron-processing core can do a certain number of neurons and synapses. We made their functionality to accelerate this computation but also to be able to emulate a lot of the convolutional neural network styles.
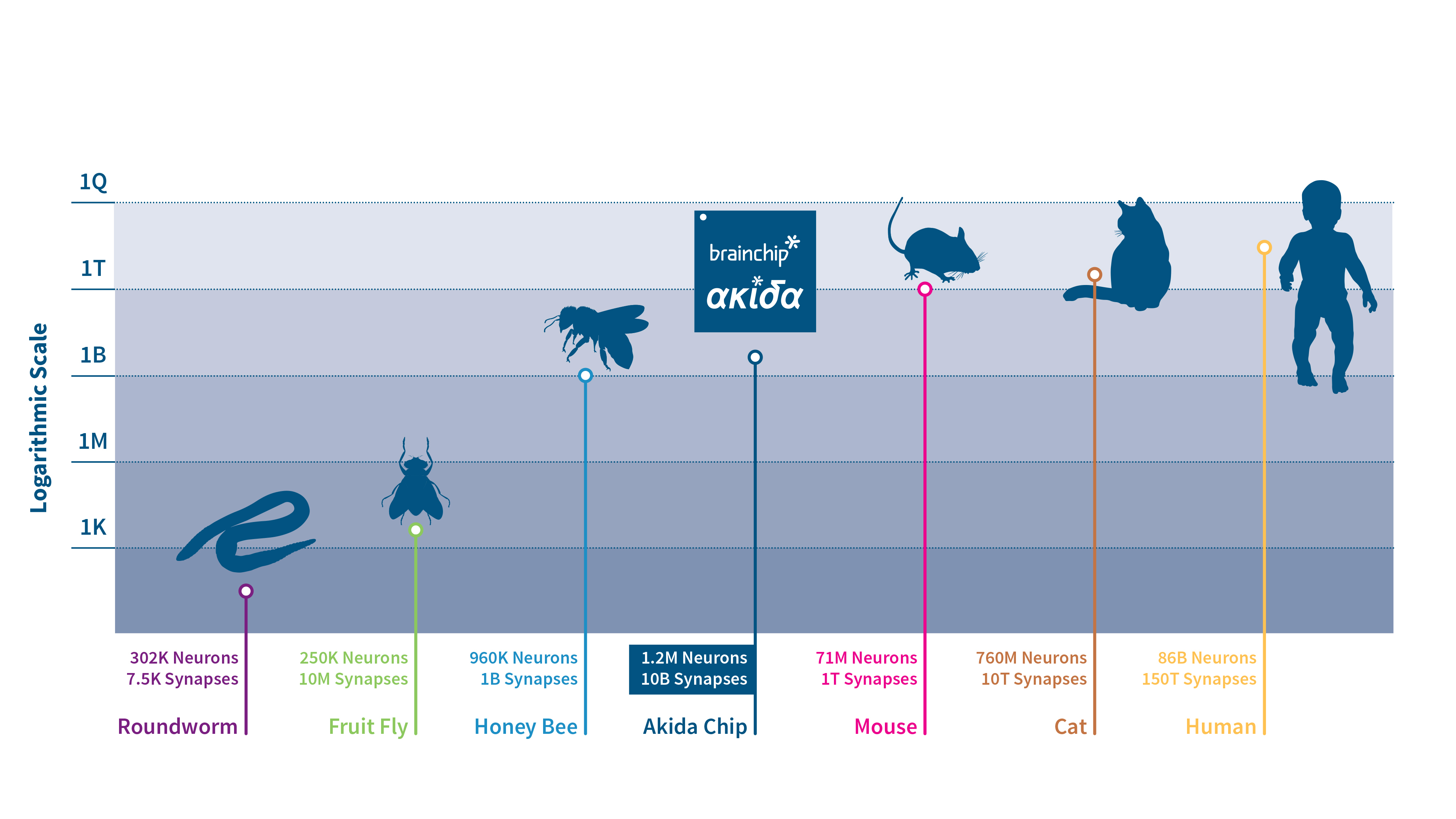
Source: BrainChip
FTCM: How do you see the future unfolding for the different kinds of hardware acceleration architecture?
RB: You’re seeing a melding of the different technologies on any given chip. If you were to look into the future, you could have an ARM processor (architecture for systems-on-chips) and an embedded FPGA for data manipulation and scaling, then you have a neuron fabric which is either accelerating SNNs and CNNs, and that all comes together in a monolithic piece of silicon.