Bravo
If ARM was an arm, BRN would be its biceps💪!
Just noticed this Tata Exlsi publication dated 3 September 2024 on multimodal AI.
I've also included a slide from Tata Exlsi's Q2 FY24 Report as a reminder of how serious they are about our partnership in terms of driving our technology into medical and industrial applications.
Publication Name: Times tech.in
Date: September 03, 2024
Multimodal AI is transforming media and communication by integrating various data types like text, images, and videos to enhance content creation, audience engagement, and advanced search capabilities. In an interview with TimesTech, Deewakar Thakyal, Senior Technology Lead at Tata Elxsi, explains how this groundbreaking technology is shaping the future of personalized and immersive content experiences across industries.
GenAI, or generative AI, is a subset of AI that can create new content, such as text, images, or code, based on patterns it learns from existing data. While GenAI can be multimodal, it’s primarily focused on generating new content. In this context, multimodal AI is focused on understanding the context, while GenAI is about creating. Multimodal AI can analyse a complex scene, such as a street intersection, and understand the interactions between pedestrians, vehicles, and traffic signals. On the other hand, GenAI can create a realistic image of a person based on a textual description.
Multimodal AI can transform content production, advertising, and creative industries. By generating cohesive and contextually relevant content across different formats, such as text, images, and audio, these models can cater to diverse needs and preferences, enhancing both reach and impact.
Additionally, multimodal AI can enable the creation of novel content formats, such as interactive storytelling or personalized product recommendations, making content more engaging and immersive. By incorporating features like speech-to-text and text-to-speech, multimodal AI can make content more accessible to a wider audience, including those with disabilities. This helps to create a more inclusive and equitable content ecosystem.
Tata Elxsi’s AIVA platform, for example, utilizes AI to create highlights of video content based on user preferences, which enables consumers to get more insights into specific parts of the video content. The use of AI-powered chatbots provides an interactive avenue for users to receive content recommendations based on their interests. Chatbots are also important support systems that answer user queries and provide content support. Keeping in mind audience demographics, multimodal AI also helps in content localisation by helping with translation and subtitling, giving a more nuanced understanding of the content to specific consumers.
This can be seen through Tata Elxsi’s content discovery and recommendation engine named uLike, which is powered by multimodal AI. The program helps users search for videos based on tags, keywords and text within videos, which helps make the content more visible. Through such mechanisms, it becomes easier to curate content that fits consumer preferences while also detecting and removing harmful or inappropriate content from platforms, which is a result of analysing user behaviour and feedback.
At the same time, while opening the scope for monetization and ethical use through licensing agreements. Multimodal AI becomes important to drive innovation in this regard.
www.tataelxsi.com
I've also included a slide from Tata Exlsi's Q2 FY24 Report as a reminder of how serious they are about our partnership in terms of driving our technology into medical and industrial applications.
BrainChip Launches Akida 2.0 Neuromorphic Edge AI Accelerator IP in "Early Access"
New edge AI accelerator IP now available, with the promise of an "order of magnitude" improvement in compute density.
www.hackster.io
Publication Name: Times tech.in
Date: September 03, 2024
Multimodal AI to Enhance Media & Communication Experiences
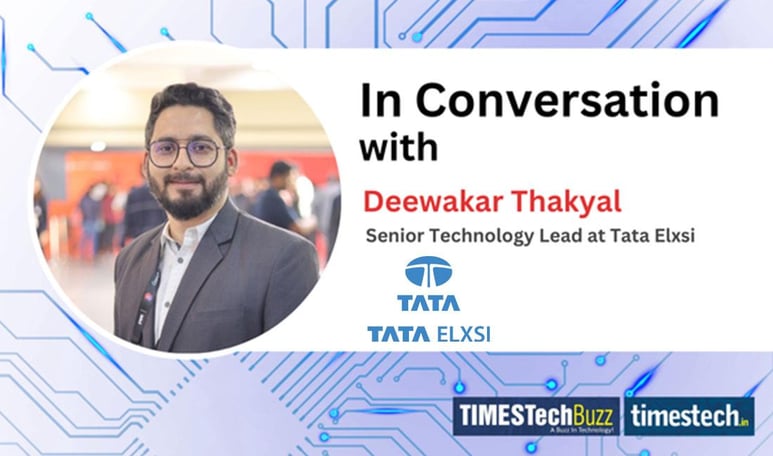
Multimodal AI is transforming media and communication by integrating various data types like text, images, and videos to enhance content creation, audience engagement, and advanced search capabilities. In an interview with TimesTech, Deewakar Thakyal, Senior Technology Lead at Tata Elxsi, explains how this groundbreaking technology is shaping the future of personalized and immersive content experiences across industries.
TimesTech: What is multimodal AI? How is it different from AI and GenAI?
Deewakar: Multimodal AI is a type of artificial intelligence that can process and understand multiple types of data simultaneously, such as text, images, audio, and video using various AI techniques, such as Natural Language Processing (NLP), Computer Vision, Speech Recognition, Machine Learning, and Large Language Models (LLMs). Unlike traditional AI, which is often limited to a single modality, multimodal AI can integrate information from different sources to provide a more comprehensive understanding of the world.GenAI, or generative AI, is a subset of AI that can create new content, such as text, images, or code, based on patterns it learns from existing data. While GenAI can be multimodal, it’s primarily focused on generating new content. In this context, multimodal AI is focused on understanding the context, while GenAI is about creating. Multimodal AI can analyse a complex scene, such as a street intersection, and understand the interactions between pedestrians, vehicles, and traffic signals. On the other hand, GenAI can create a realistic image of a person based on a textual description.
TimesTech: How is multimodal AI enhancing content creation?
Deewakar: Multimodal AI is revolutionizing content creation by allowing for more dynamic, engaging, and personalized experiences. It enhances understanding by processing various forms of content simultaneously, tailors content to individual needs, assists human creators, enables new content formats, and improves accessibility. For example, multimodal AI can analyse user preferences and behaviour to create personalized recommendations, suggesting products or articles that align with their interests. It can also assist human creators by generating ideas, suggesting different angles, or providing feedback on drafts.Multimodal AI can transform content production, advertising, and creative industries. By generating cohesive and contextually relevant content across different formats, such as text, images, and audio, these models can cater to diverse needs and preferences, enhancing both reach and impact.
Additionally, multimodal AI can enable the creation of novel content formats, such as interactive storytelling or personalized product recommendations, making content more engaging and immersive. By incorporating features like speech-to-text and text-to-speech, multimodal AI can make content more accessible to a wider audience, including those with disabilities. This helps to create a more inclusive and equitable content ecosystem.
TimesTech: What is the role of Multimodal AI in improving audience engagement?
Deewakar: With multimodal AI comes the integration of various types of data such as text, images, videos etc. With such varied content, the use of AI makes it easy to ascertain user preferences by processing multiple sensory inputs simultaneously. With consumers looking for more personalization in content and more digital platforms struggling to keep up with the demand, employing multimodal AI helps enhance audience engagement by directly making use of audience insights.Tata Elxsi’s AIVA platform, for example, utilizes AI to create highlights of video content based on user preferences, which enables consumers to get more insights into specific parts of the video content. The use of AI-powered chatbots provides an interactive avenue for users to receive content recommendations based on their interests. Chatbots are also important support systems that answer user queries and provide content support. Keeping in mind audience demographics, multimodal AI also helps in content localisation by helping with translation and subtitling, giving a more nuanced understanding of the content to specific consumers.
TimesTech: Does multimodal AI help in advanced search and analysis? How?
Deewakar: Multimodal AI can be extended to provide video insights like facial expressions, and situational sentiments as well as identify actions and objects by integrating and analysing data from multiple sources such as images, audio, text etc., which becomes helpful for consumers to get a better understanding of the content. Multimodal AI is extensively utilized by advertisers and media companies to deliver personalized ads that fit user behaviour and are optimized for different platforms like websites, mobile apps, and social media.This can be seen through Tata Elxsi’s content discovery and recommendation engine named uLike, which is powered by multimodal AI. The program helps users search for videos based on tags, keywords and text within videos, which helps make the content more visible. Through such mechanisms, it becomes easier to curate content that fits consumer preferences while also detecting and removing harmful or inappropriate content from platforms, which is a result of analysing user behaviour and feedback.
At the same time, while opening the scope for monetization and ethical use through licensing agreements. Multimodal AI becomes important to drive innovation in this regard.
TimesTech: What is the futuristic scope of multimodal AI?
Deewakar: With major digital transformation firms inching toward multimodal AI, it only goes to show that this will be a major breakthrough in content generation and personalisation across the media and entertainment industry. However, this technology can be extended to other industries as well, such as e-commerce, healthcare, education etc. Due to the significance of technologies like NLP, which can better analyse context and sentiment, there is a higher scope for multimodal AI to enhance the human-machine experience. However, it also becomes necessary to pay attention to ethical concerns and privacy issues with its use, as this involves analysing user data to provide insights. With the proper measures, multimodal AI will be transformational for the industry and can bring in the much-needed innovation, as promised.Tata Elxsi - Multimodal AI to Enhance Media & Communication Experiences
Tata Elxsi
