Dallas
Regular
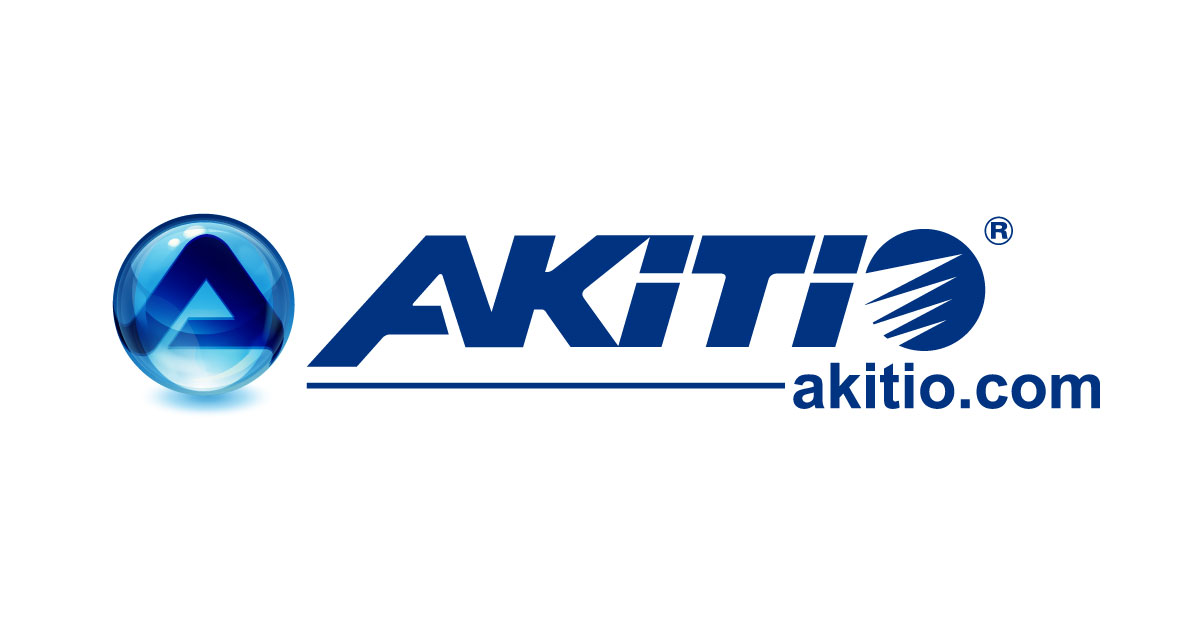
AKiTiO eGPU over Thunderbolt 3, PCIe Expansion, RAID Storage
Akitio designs and produces Thunderbolt 3 PCIe expansion boxes for external GPUs (eGPU), 10GbE Ethernet and other add-on cards and external RAID storage drives.
I forgot what GREEN looks like!
BRCHF today closed at USD$0.7650 (+4.79%)
Tomorrow is another presentation for BrainChip. Will we see a new LOGO displayed? Possibly, although I’m not holding my breath.
Onward and upward!
Morning Miaeffect,View attachment 2343
I am wearing my special glasses today. Its not just my ASX, everything is red to me equally. Yeah I feel better now.
I beat you. Na na ni na na![]()
BrainChip Continues Expansion with Sales Partnerships in New Geographic Markets
BrainChip Continues Expansion with Sales Partnerships in New Geographic Marketswww.businesswire.com
![]()
BrainChip Continues Expansion with Sales Partnerships in New Geographic Markets
BrainChip Continues Expansion with Sales Partnerships in New Geographic Marketswww.businesswire.com
I have already given this same shout out to the 1,000 Eyes who disclosed this relationship last year between Eastronics and Brainchip. Is knowing something four months before the general market considered insider trading??? Well done 1,000 Eyes keep the news flowing.
Drawing on some further background information that may interest you and others.Hi FF,
Naaaa no insider trading, just lots of eyes doing great research.
It's funny when I was saving the partner logo's screenshot just before on my computer, I was saving it as eastronics & as I was typing the file name to save it, I realized I already had 4 previously saved screenshots named eastronics 1 2 3 & 4 & I checked the date I saved them & it was June last year, so we must have all been talking about it then at that other place.
This is fantastic confirmation of what we already knew & is opening us up to the huge Israel & Europe markets.
Goooooooo BrainChip![]()
Drawing on some further background information that may interest you and others.
Last year it was disclosed not long after the presentation by a certain small engineering firm on a Rob Telson Brainchip podcast that in furtherance of the ARM business model he was setting up Brainchip’s own list of Engineering firms.
If you go to ARM’s website they have a list of these small engineering firms which the last time I looked numbered about 34.
For those who do not know what these guys do it is two fold.
Customers come to them with a problem say my underpants keep riding up but there is nothing in the market presently that will overcome the problem. These firms then go about trying to come up with a solution and sourcing products from their partner firms and modifying them to solve the customers problem.
The second way they make a buck is by going out into the world and trying to identify potential customers suffering from a similar or different problem with their underpants and coming up with a solution with there partners and selling it to these potential customers.
I think it was the former CEO Mr. Dinardo who used the term when describing these relations as great because these guys “have to hunt and kill there own”. In other words it does not cost Brainchip anything to have these relationships.
The thing is though to get your name on their lists is not easy for this very same reason. They need to believe that what you have will add value and be something they can sell otherwise they do not eat.
Eastronics and Saleslink are a big deal for this reason and where Eastronics is concerned it was suggested they may have been the link between Brainchip and NaNose.
Following the ARM model there will be more announcements in this area and probably quite soon. I know for a fact that Rob Telson was run off his feet covering both sales and marketing and was finding it difficult to get the time to close out all of these sales relationships with his target small engineering firms.
My opinion only DYOR
FF
AKIDA BALLISTA
Same here TechGirl I did manage to buy some with my Super and been topping up since SP keeping sliding since our all time high. but now running out of just like that Gif you postedGod its so much fun watching this all play out, just wish I had more cash or Super to invest
![]()
Hi FF,Drawing on some further background information that may interest you and others.
Last year it was disclosed not long after the presentation by a certain small engineering firm on a Rob Telson Brainchip podcast that in furtherance of the ARM business model he was setting up Brainchip’s own list of Engineering firms.
If you go to ARM’s website they have a list of these small engineering firms which the last time I looked numbered about 34.
For those who do not know what these guys do it is two fold.
Customers come to them with a problem say my underpants keep riding up but there is nothing in the market presently that will overcome the problem. These firms then go about trying to come up with a solution and sourcing products from their partner firms and modifying them to solve the customers problem.
The second way they make a buck is by going out into the world and trying to identify potential customers suffering from a similar or different problem with their underpants and coming up with a solution with there partners and selling it to these potential customers.
I think it was the former CEO Mr. Dinardo who used the term when describing these relations as great because these guys “have to hunt and kill there own”. In other words it does not cost Brainchip anything to have these relationships.
The thing is though to get your name on their lists is not easy for this very same reason. They need to believe that what you have will add value and be something they can sell otherwise they do not eat.
Eastronics and Saleslink are a big deal for this reason and where Eastronics is concerned it was suggested they may have been the link between Brainchip and NaNose.
Following the ARM model there will be more announcements in this area and probably quite soon. I know for a fact that Rob Telson was run off his feet covering both sales and marketing and was finding it difficult to get the time to close out all of these sales relationships with his target small engineering firms.
My opinion only DYOR
FF
AKIDA BALLISTA
Not sure if anyone else has had a chance to watch this webinar from this morning, but it was actually really interesting and entertaining is some parts. I’d recommend having a listen if you’ve got time.
View attachment 2016
Ralph Etienne-Cummings directs the Computational Sensory-Motor Systems Laboratory at Johns Hopkins University. His research spans a range of electrical and computer engineering topics. Including, but not limited to, mixed-signal VSLI systems, computational sensors, computer vision, neuromorphic engineering, smart structures, mobile robotics, and neuroprosthetic devices. His research has convinced him of the need for neuromorphic computing.
![]()
DP0664909 - Biomimetic Ultra-Thin Compound-Eye Vision Sensor
research-repository.uwa.edu.au
Etienne-Cummings is associated with UWA