equanimous
Norse clairvoyant shapeshifter goddess
Not sure about all the Chinese connections
So will we be getting royalties for these
Speck is a fully event-driven neuromorphic vision SoC. Speck is able to support large-scale spiking convolutional neural network (sCNN) with a fully asynchronous chip architecture. Speck is fully configurable with the spiking neuron capacity of 320K. Furthermore, it integrates the state-of-art dynamic vision sensor (DVS) that enables fully event-driven based, real-time, highly integrated solution for varies dynamic visual scene. For classical applications, Speck can provide intelligence upon the scene at only mWs with a response latency in few ms.
100-1000X lower
Ultra-low latency
End-end latency <5ms, reaction speed up 10-100x
Privacy security
Processing of visual applications based on dot matrix data
Cutting-edge algorithm
Various sCNN algorithms
So will we be getting royalties for these
SPECK
The world’s first fully event-driven neuromorphic vision SoC using the dynamic vision sensing and the spiking neural network technology.Speck is a fully event-driven neuromorphic vision SoC. Speck is able to support large-scale spiking convolutional neural network (sCNN) with a fully asynchronous chip architecture. Speck is fully configurable with the spiking neuron capacity of 320K. Furthermore, it integrates the state-of-art dynamic vision sensor (DVS) that enables fully event-driven based, real-time, highly integrated solution for varies dynamic visual scene. For classical applications, Speck can provide intelligence upon the scene at only mWs with a response latency in few ms.
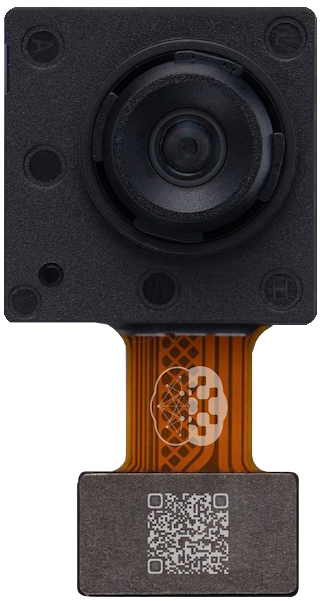
Applications
Smart Toy- Gesture control
- Smart tracking
- Gesture control
- Fall detection
- Approaching detection
- Lane detection
- Sign recognition
- Driver attention tracking
- Obstacle detection
- Obstacle detection
- Object tracking
100-1000X lower
Ultra-low latency
End-end latency <5ms, reaction speed up 10-100x
Privacy security
Processing of visual applications based on dot matrix data
Cutting-edge algorithm
Various sCNN algorithms