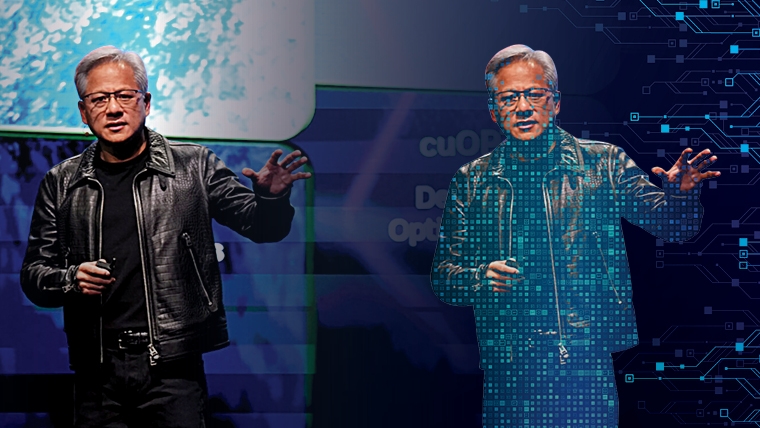
Raising the Stakes for Accelerated Computing
A Booz Allen interview with NVIDIA founder and CEO Jensen Huang about the future of AI and computing.
He bought back just to sell right after… watch and see
He's a she.He bought back just to sell right after… watch and see
Looks like "They" whoever they are has decided its time to buy again.![]()
I think both Dolci and Ariel have made handsome profits trading BRN over the years. Dolci generally promoting lows to buy in, then pumping till she sells out and then rinse repeating.He bought back just to sell right after… watch and see
Makes things not better with this behaviour. Just my opinion .. no financial adviceHe's a she.
I have no problem with how someone makes his money. My problem is when people influence others just to make a profit. While some have a plan, others have no clue and end up losing their investment. The fact that it’s women doing this doesn’t make it any better. It’s strange that the majority of people using such practices are women. Is T&J also a woman? I wouldn’t be surprised in that case. (Of course, there are amazing women like Bravo.)I think both Dolci and Ariel have made handsome profits trading BRN over the years. Dolci generally promoting lows to buy in, then pumping till she sells out and then rinse repeating.
Ariel mercilessly shorted the stock a few years ago and probably made good bank.
Now that the price is in the gutter they have changed their tune and relentlessly pump hoping to ride the wave back up.
Neither give a rats about the company or what it’s trying to achieve or the impact it’s success may have upon the world.
And that’s ok.
They have a purely mercantile relationship to the company.
Best served over at the cesspit that is the crapper.
Generally the contributors here tend to take a longer term and more comprehensive view whilst still of course looking for a positive outcome valuation wise.
I have come to the view that a mixture of the approaches is probably the best with separate long term and trading parcels to take advantage of both strategies.
Working towards that scenario now, but wish I had implemented it a few years back.
GLTAH
Goodness me! What have we here?!!!!
The below article includes quotes from Steven Meier of the Naval Research Centre.The importance of what this might mean to us can be further ascertained in light of the collaboration between the AFRL and the NRL, as is outlined in this extract from the 2023 Space Symposium..
EXTRACT
View attachment 77166
![]()
AFRL showcases key programs at 2023 Space Symposium
The Air Force Research Laboratory, or AFRL, will feature several space-related technologies and programs during the 38th Space Symposium at the Broadmoor, Colorado Springs, Colorado, April 17-20, 2023.www.dvidshub.net
View attachment 77161
View attachment 77160
Pentagon
Power generation challenges could overshadow Stargate AI initiative
By Courtney Albon
Saturday, Jan 25, 2025
President Donald Trump and AI industry leaders and investors on Tuesday unveiled a five-year, $500 billion initiative to boost AI development in the U.S. (Julia Demaree Nikhinson/AP)![]()
While the Defense Department is likely to benefit from OpenAI’s announcement this week that it would invest half a trillion dollars to build new artificial intelligence data centers around the country, Pentagon officials warned that the U.S. lacks the energy resources and computing power to support the new infrastructure — and solving that problem won’t be easy.
OpenAI announced the project, dubbed Stargate, on Tuesday, pledging an initial $100 billion — plus another $400 billion over the next five years — to build new AI infrastructure across the U.S. and create “hundreds of thousands of American jobs” in the process. Early funders include Softbank, OpenAI, Oracle and MGX — a technology investment firm based in the United Arab Emirates — and OpenAI will partner with Oracle, Microsoft, Arm and NVIDIA on technology development.
During a press conference Tuesday at the White House, President Donald Trump called the effort a “monumental undertaking” and said the White House would support the project, in part, through issuing emergency declarations, though he didn’t expand on details.
The Defense Department has an ambitious vision for using AI across a range of military missions, including data collection, intelligence analysis, campaigning and logistics. But running those tools and applications takes more computing power and space than DOD has access to.
Roy Campbell, deputy director of advanced computing in the Office of the Undersecretary of Defense for Research and Engineering, said Thursday that many times, bases outside of the U.S. don’t have the computing power they need to retrain new AI tools.
“In some cases, for you to be able to handle a situation a forward operating base can’t handle, you have to kick that back to [the continental United States] and use the DOD supercomputing centers that we have there,” he said during a panel at the Potomac Officers Club’s annual Research and Development Summit in McLean, Virginia.
Jeff Waksman, who’s leading an effort in the Pentagon’s Strategic Capabilities Office to develop a mobile nuclear reactor, said the strain that technologies like AI and high-power computing place on the electric grid raises questions about who should have access to data and how to mitigate the risk of blackouts.
“This is not a problem that industry or the DOD can figure out by itself. It’s about the nation’s grid as a whole,” said Waksman, who spoke on a panel with Campbell. “It’s probably the most underrated challenge of this huge $500 billion announcement.”
Waksman’s nuclear reactor program, known as Project Pele, offers one answer to that challenge: using nuclear power to source energy for AI computing.
The effort, initiated in 2019, aims to demonstrate the first-ever U.S. prototype of a portable nuclear reactor within five years. The mobile reactor, which the department estimates could deliver one to five megawatts of electrical power over a minimum three-year operating life, would support DOD’s growing energy needs by providing power to austere locations.
The Pentagon broke ground at the Project Pele test site at the Idaho National Laboratory last September and plans to begin assembling the reactor — built by BWXT Advanced Technologies — as soon as next month. The department aims to demonstrate the technology in 2026.
“It’s going to be the first generation portable nuclear reactor built anywhere in the world, outside of China,” Waksman said. “It’s very much not a paper project anymore.”
View attachment 77162 View attachment 77162
Another potential solution to the AI power problem is making processors more effective at crunching data. Steven Meier, associate director of space technology at the Naval Research Center, said his lab is exploring the use of more efficient neuromorphic processors that can be 100 times more efficient than a standard processor. Essentially, neuromorphic processors take up less space, work faster and use less energy.
“There’s huge gains to be made in terms of neuromorphic processors making AI and [machine learning] more accessible on autonomous vehicles of all shapes and sizes,” Meier said at the conference.
![]()
Power generation challenges could overshadow Stargate AI initiative
Pentagon officials said the U.S. lacks the energy resources and computing power to support the new infrastructure — and solving that problem won’t be easy.www.defensenews.com
Hi Fur.I have no problem with how someone makes his money. My problem is when people influence others just to make a profit. While some have a plan, others have no clue and end up losing their investment. The fact that it’s women doing this doesn’t make it any better. It’s strange that the majority of people using such practices are women. Is T&J also a woman? I wouldn’t be surprised in that case. (Of course, there are amazing women like Bravo.)
That is what he wants you to believe. It's all part of his trading strategy.He's a she.