buena suerte :-)
BOB Bank of Brainchip
looking like Battle of the Bots this arvo ......
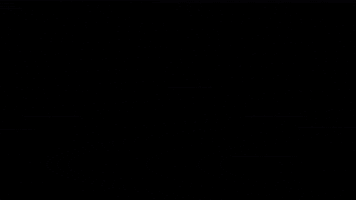
A few more goes at the $1.20 should see us heading to the next resistance at $1.25looking like Battle of the Bots this arvo ......![]()
Looking that wayA few more goes at the $1.20 should see us heading to the next resistance at $1.25
I have a suspicion the large 1.250 order will disappear.A few more goes at the $1.20 should see us heading to the next resistance at $1.25
This guy epitomises BRN today.
![]()
Researches Use IoT for Cancer Diagnosis
Artificial neurons and an AI system are being used to assess benign or malignant tumors
- Written by Scarlett Evans
- 14th July 2022
Researchers from the Korea Institute of Science and Technology (KIST) have developed a novel cancer diagnosis technology; a simple but accurate method that uses tactile neuron devices combined with AI technology.
Typically, a non-invasive method of diagnosis is ultrasound elastography; however, interpretation of the results can vary. The new method identifies and measures the stiffness and distribution of a tumor, allowing for accurate cancer diagnosis.
The KIST team developed this alternative method to improve accuracy and speed up the time of prognosis. For their experiments, the team combined tactile neuron devices with artificial neural network learning methods, applying pressure to a potentially cancerous site, with the pressing force generating electrical spikes that increase or decrease depending on the stiffness of the object encountered.
The method falls under the category of “neuromorphic technology,” a data processing technology that has become increasingly popular given its compatibility with AI, IoT and autonomous technologies. It seeks to emulate the human brain’s method of processing vast amounts of information using minimal energy, with neurons receiving external stimuli through sensory receptors which are then converted into electrical spike signals.
Deploying this for disease diagnosis, the team used elastography images of malignant and benign breast tumors in combination with a spiking neural network learning method. The pixels from the color-coded ultrasound elastography image correlated to the stiffness of the object encountered and were converted to a frequency value to train the AI.
Following this process, the team reported a breast tumor diagnosis accuracy of 95.8%, saying the developed artificial tactile neuron technology is capable of “detecting and learning mechanical properties with a simple structure and method.”
The team also anticipated the device could be used in low-power and high-accuracy disease diagnosis and applications such as robotic surgery, where a surgical site needs to be quickly determined with minimal to no human interaction.
![]()
Researches Use IoT for Cancer Diagnosis
Artificial neurons and an AI system are being used to assess benign or malignant tumorswww.iotworldtoday.com
Using Akida?
![]()
Researches Use IoT for Cancer Diagnosis
Artificial neurons and an AI system are being used to assess benign or malignant tumors
- Written by Scarlett Evans
- 14th July 2022
Researchers from the Korea Institute of Science and Technology (KIST) have developed a novel cancer diagnosis technology; a simple but accurate method that uses tactile neuron devices combined with AI technology.
Typically, a non-invasive method of diagnosis is ultrasound elastography; however, interpretation of the results can vary. The new method identifies and measures the stiffness and distribution of a tumor, allowing for accurate cancer diagnosis.
The KIST team developed this alternative method to improve accuracy and speed up the time of prognosis. For their experiments, the team combined tactile neuron devices with artificial neural network learning methods, applying pressure to a potentially cancerous site, with the pressing force generating electrical spikes that increase or decrease depending on the stiffness of the object encountered.
The method falls under the category of “neuromorphic technology,” a data processing technology that has become increasingly popular given its compatibility with AI, IoT and autonomous technologies. It seeks to emulate the human brain’s method of processing vast amounts of information using minimal energy, with neurons receiving external stimuli through sensory receptors which are then converted into electrical spike signals.
Deploying this for disease diagnosis, the team used elastography images of malignant and benign breast tumors in combination with a spiking neural network learning method. The pixels from the color-coded ultrasound elastography image correlated to the stiffness of the object encountered and were converted to a frequency value to train the AI.
Following this process, the team reported a breast tumor diagnosis accuracy of 95.8%, saying the developed artificial tactile neuron technology is capable of “detecting and learning mechanical properties with a simple structure and method.”
The team also anticipated the device could be used in low-power and high-accuracy disease diagnosis and applications such as robotic surgery, where a surgical site needs to be quickly determined with minimal to no human interaction.
![]()
Researches Use IoT for Cancer Diagnosis
Artificial neurons and an AI system are being used to assess benign or malignant tumorswww.iotworldtoday.com
I found an interesting article/interview published a few days ago with Jean-Rene Leuepeys who is the deputy director and CTO at CEA-Leti. He says they are preparing the next generation of their own neuromorphic chip which will have "more than 100,000 neurons on a chip and more than 75 million synapses".I'm thinking Jean-Rene must have missed the memo about AKIDA and it's 1.2 million neurons and 10 billion synapses.
View attachment 10599
CEA-Leti and the Silicon Valleys of Grenoble, France | Computer Weekly
At the intersection of three valleys in the French Alps lies the city of Grenoble, a hotbed of microelectronics, where much of the innovation is fuelled by CEA-Leti.www.computerweekly.com
since Qualcomm is a Brainchip customer, I have a hard time assuming Brainchip is involvedIt wouldn't surprise me if we were involved in this in some way, shape or form.
Ericsson, Thales, global leader in Aerospace, Defence, Security & Digital Identity, and wireless technology innovator Qualcomm Technologies, Inc. are planning to take 5G out of this world and across a network of Earth-orbiting satellites.
View attachment 13685 /ATTACH]
View attachment 13683
![]()
Ericsson, Qualcomm and Thales to take 5G into space IT Voice | IT in Depth
Ericsson (NASDAQ: ERIC), Thales (Euronext Paris: HO), global leader in Aerospace, Defence, Security & Digital Identity, and wireless technology innovator Qualcomm Technologies, Inc. (NASDAQ: QC…www.itvoice.in
since Qualcomm is a Brainchip customer, I have a hard time assuming Brainchip is involved![]()
ASX need to do their fucking job, goes for both buy and sellAfternoon Chippers,
Our mystery manipulator has dropped their sell order .
700,515 units for $1.195
Esq.
Afternoon Gex,ASX need to do their fucking job, goes for both buy and sell