M_C
Founding Member
Hey all,
New Qualcomm thread.............think we're going to need it. To kick it off I came across this interesting article linking Qualcomm to a few car manufactures we have been linking to BRN including Toyota, Honda and Mercedes...............
Nothing definitive here but given everything we have on Qualcomm to date, I'd say there is a favourable connection here..............after all "we see these companies as partners not competitors...." Right?
"The key is that Qualcomm is not going it alone, announcing partnerships with companies including Honda, Volvo and Renault, noting that it is also working with most major automakers, including Mercedes-Benz, BMW, Toyota and Chevrolet."
www.iotworldtoday.com
This article talks also about Qualcomms recent partnership with Ferrarri
www.theverge.com
New Qualcomm thread.............think we're going to need it. To kick it off I came across this interesting article linking Qualcomm to a few car manufactures we have been linking to BRN including Toyota, Honda and Mercedes...............

Nothing definitive here but given everything we have on Qualcomm to date, I'd say there is a favourable connection here..............after all "we see these companies as partners not competitors...." Right?
"The key is that Qualcomm is not going it alone, announcing partnerships with companies including Honda, Volvo and Renault, noting that it is also working with most major automakers, including Mercedes-Benz, BMW, Toyota and Chevrolet."

Qualcomm Drives to Platforms for In-Car Innovations
The future of automotive will prove more and more connected things in a vehicle will be required to work together, much of it in real time
This article talks also about Qualcomms recent partnership with Ferrarri
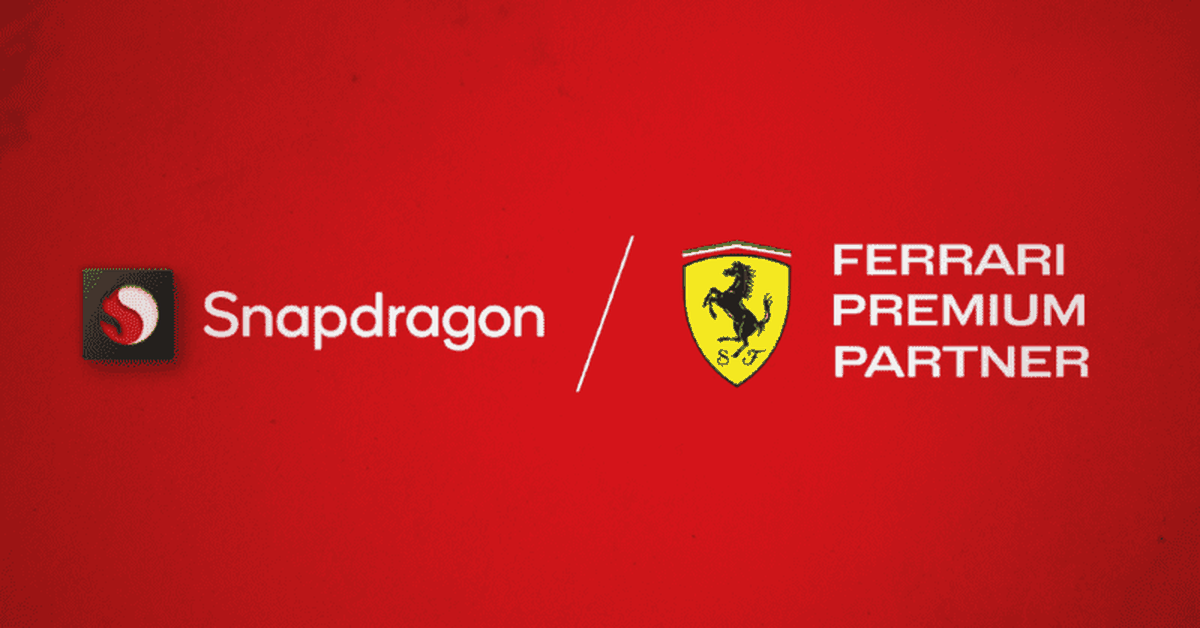
Ferrari is Qualcomm’s latest partner to build smarter cars
Qualcomm is working with another major car company.
Last edited: