Fullmoonfever
Top 20
Dell Technologies , Internal use - confidential , copyright 2021 . Don’t worry , you’re secret is safe with me , I won’t show anyone …..
View attachment 5865
Nice @Doz
Does tie in as well with prev Dell post I did in the Gen Article thread mid Mar.
We get our mention about 3/4 down in red.
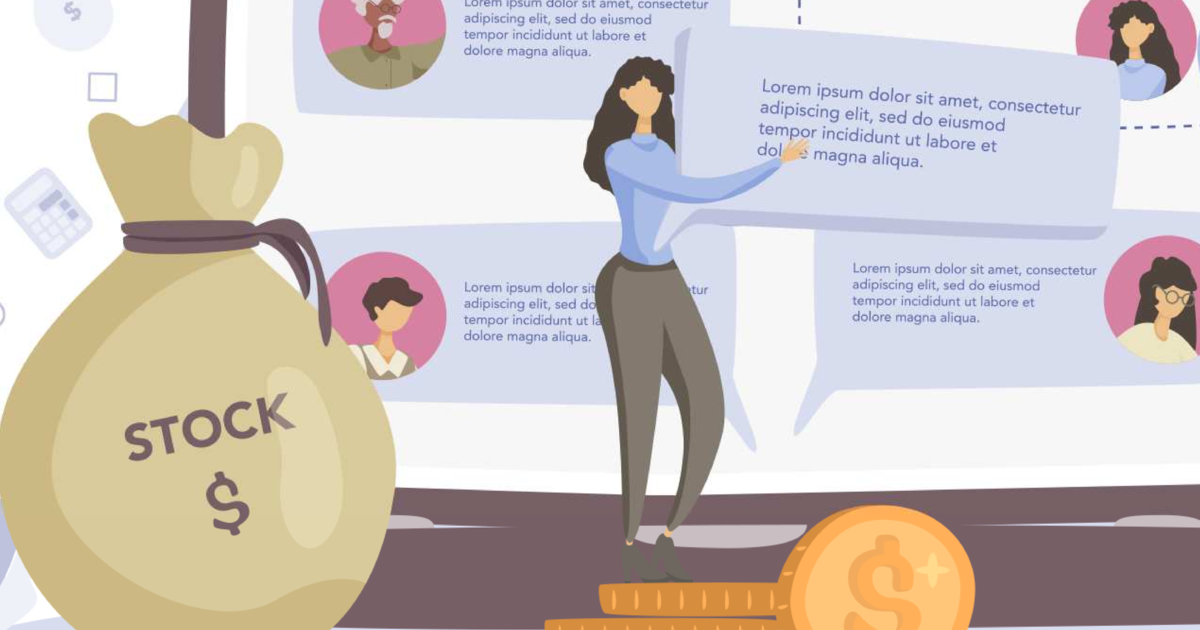
Breaking News- new BRN articles/research
Some may remember reading a post I put up regarding LinkedIn followers liking brainchip. One of them was high up in the Telstra Purple divison. I just came across this. https://www.itnews.com.au/news/telstra-to-drive-ai-into-every-part-of-its-business-576771


人工智能未来发展之路
作者:贾真博士 戴尔科技集团中国研究院 总监人工智能(Artificial Intelligence,AI)已经无处不在。在数字化转型的时代,当人们转向更加频繁的数字交互时,来自数字虚拟世界的海量数据与真实物理世界无缝融合。 …
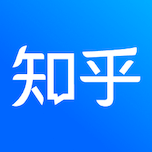
The future of artificial intelligence

Dell Technologies
Verified account
3 people liked this article
content
put away
Problems that need to be solved by artificial intelligence
The future of artificial intelligence
Author: Dr. Jia Zhen, Director of Dell Technologies China Research Institute
Artificial Intelligence (AI) is already ubiquitous. In the era of digital transformation, when people turn to more frequent digital interactions, the massive data from the digital virtual world seamlessly merges with the real physical world. As the amount, variety, and speed of data generation increases, AI represents an important critical step in extracting insights from massive amounts of data and advancing other emerging technologies .AI algorithms and hardware-accelerated systems are improving business decision-making efficiency, improving business processes, and delivering smarter, more real-time data analysis results at scale. AI is fundamentally changing the way businesses operate, redefining the way people work, and transforming industries on a global scale. In the era of digital transformation, our society and enterprises need to make more use of intelligent information system architecture, application software and algorithms, and data-first strategies to fully realize the business potential of enterprises.
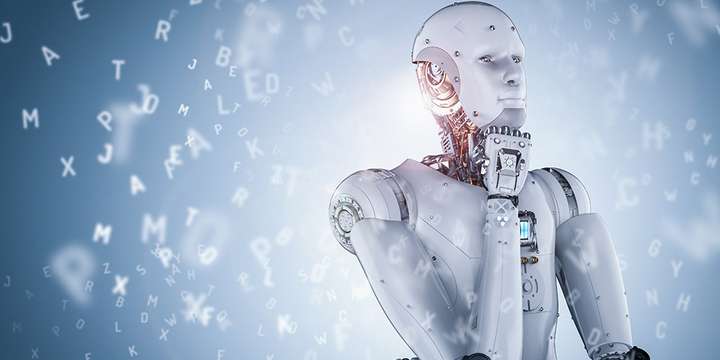
Here I also briefly list some key figures reflecting the booming development of artificial intelligence: 62% of global enterprises have invested in artificial intelligence to some extent[1]; 53% of global data and analytics decision makers say they are planning to implement some artificial intelligence in the form of [2]; by 2022, 75% of enterprises will embed intelligent automation into technology and process development [3].
As mentioned above, artificial intelligence has made great progress in recent years, but we still have many problems that need to be solved urgently. In this article, I will first analyze the core problems that remain to be solved in the current development of artificial intelligence, and then propose some ideas for our key development directions in the field of artificial intelligence.
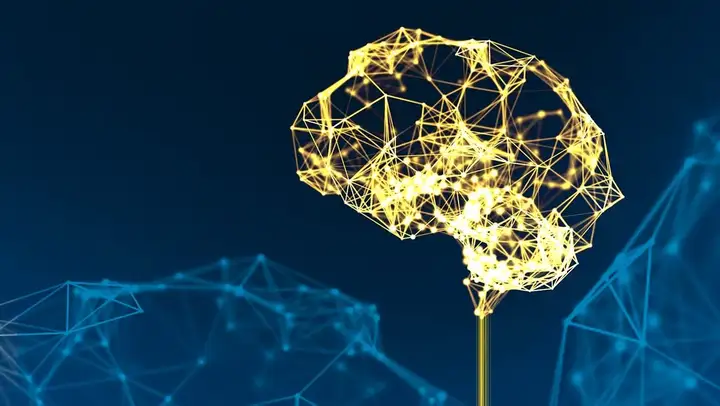
Problems that need to be solved by artificial intelligence
- Algorithmic complexity of artificial intelligence: The mainstream algorithms of artificial intelligence today are based on the Deep Neural Network [13] of Machine Learning. With the development of artificial intelligence technology, the structure of deep neural network is becoming more and more complex, and there are more and more hyperparameters. Sophisticated deep neural networks improve the accuracy of machine learning models, but configuring and debugging such complex networks can be prohibitive for ordinary users of artificial intelligence. The ease of development, debugging, and deployment of deep neural network algorithms and applications is also becoming more and more urgent .
- Data scarcity of artificial intelligence: The efficient reasoning and recognition of deep neural networks nowadays mainly depends on the support of a large amount of training data. Open databases such as ImageNet [9] provide thousands of images, videos and corresponding annotation information. Through the training of a large amount of data, the machine learning model can almost cover the changes of various reasoning and recognition scenarios. However, if the amount of data is not enough or the type is not comprehensive enough, the performance of the machine learning model is bound to be limited. In the application of artificial intelligence in industry, the problem of data shortage is particularly prominent. Different from traditional reasoning and recognition applications for ordinary consumers, artificial intelligence applications in the industry are often unique business-related problems (such as: intelligent manufacturing, remote system debugging and maintenance, etc.), corresponding data (especially negative samples) Very few. In the case of shortage of training data, how to improve the algorithm model of artificial intelligence so that it can still work efficiently under specific scenarios and limited data is also a new and urgent task .
- High computational consumption of artificial intelligence: As mentioned in the previous two aspects, the complexity of deep neural networks and the diversity of big data will lead to the high consumption of computing resources in current artificial intelligence applications. At present, the training of more advanced machine learning models, such as GPT-3, takes several months to utilize high-performance clusters [10]. Ordinary machine learning models can take hours or even days to train on traditional x86 high-performance servers if the amount of data is large. At the same time, when the trained model performs inference and recognition tasks, due to the complex model structure, many hyperparameters, and complex calculations, the requirements for computing resources of terminal devices that process data are also higher. For example, lightweight IoT devices cannot run complex machine learning inference and recognition models, or for smart terminal devices, such as smartphones, running complex machine learning models will lead to large battery consumption. How to better and fully optimize computing resources to support machine learning training and inference recognition is also another new urgent task.
- Interpretability of artificial intelligence: Artificial intelligence technology using deep neural networks, due to the complexity of neural networks, many times people treat them as a "black box". The user inputs the data that needs to be recognized by reasoning, and the deep neural network obtains the result of reasoning and recognition through a series of "complex and unknown" mathematical processing. However, we cannot intuitively analyze why the input data will get the corresponding results through the complex neural network. In some key AI areas, such as autonomous driving, the interpretability of AI decisions is critical . Why does an automated driving system make such a driving decision in some critical safety-related scenarios? Why is the reasoning and recognition of road conditions sometimes wrong? These inference and identification conclusions from the "black box" must be interpretable and must be traceable. Only when artificial intelligence can be explained can we find the basis for decision-making and judgment and find out the reason for the error of reasoning and identification. "From effect to cause", we can improve the performance of deep neural network, so that it can provide artificial intelligence applications more efficiently, safely and reliably in different occasions .
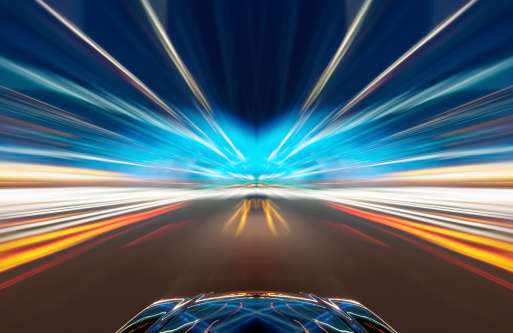
The future of artificial intelligence
In view of the four major problems that artificial intelligence needs to solve urgently listed above, I will briefly describe the main technical directions that we need to pay attention to for future development:- First, we need to be facilitators of the “3rd Wave AI”, preparing our corporate society for the coming AI revolution. These changes will drive our data management, artificial intelligence algorithms, and hardware accelerators to flourish. We need to actively develop new models of collaboration with clients and research entities driving the “third wave of AI.” So, what is the "third wave of artificial intelligence"?
- From an algorithmic point of view, we summarize it as the concept of Contextual Adaptation. Specifically, we need to pay more attention to the following algorithm development trends:
- We need to establish reliable decision-making capabilities in artificial intelligence systems, so that people can understand or analyze why the "black box" machine learning algorithm model makes inference and identification decisions. Specifically, there are three problems that need to be solved for safe and reliable artificial intelligence: boundary problem, backtracking problem and verifiable problem . We call such a capability “AI explainability” [5].
- How to build AI systems that can train machine learning models with one (One-Shot Learning [6]) or very few (Few-Shot Learning [7]) examples. As mentioned above, in real industrial application scenarios, data is relatively scarce. Effectively constructing and training machine learning models under extremely limited data is a hot research direction at present .
- Compared with the traditional and open-loop offline learning (Offline Learning), online learning (Online Learning) [20], as an emerging direction, is a closed-loop system: the machine learning model sends the inference and recognition results to the user based on the current parameters and architecture, User feedback is collected and used to update the optimization model, thus completing an optimization process that continuously receives information and updates iteratively. In other words, machine learning models need to dynamically accept sequential data and update themselves to optimize performance .
- Multi-Task Learning [21] refers to a learning method in which the training data contains samples from multiple different scenes, and the scene information is used to improve the performance of machine learning tasks during the learning process. The scene adaptation method in traditional transfer learning usually only realizes the bidirectional knowledge transfer between the original scene and the target scene, while multi-scene task learning encourages the bidirectional knowledge transfer between multiple scenes .
- The machine learning model is trained based on the contextual information of the context. With the passage of time and the migration of the scene, the artificial intelligence system will gradually learn the method of constructing the updated model autonomously [11]. Machine learning models derived from contextual learning (Contextual Learning [15]) will be used to better perceive the world and help humans make inference decisions more intelligently .
- With the rapid development of artificial intelligence technology, knowledge representation and knowledge reasoning based on deep neural networks have received more and more attention, and scene knowledge graphs of different scenarios have appeared one after another [22]. As a semantic network, the scene knowledge graph depicts scene knowledge and provides the basis for inference and recognition tasks within the scene. As an application of knowledge reasoning, the question answering system based on knowledge graph has made great progress .
- Machine learning models derived from contextual learning can also help us better abstract our data and the world we need to perceive [16], thereby making our artificial intelligence systems more generalized and adaptable Solve all kinds of complex problems.
- From an algorithmic point of view, we summarize it as the concept of Contextual Adaptation. Specifically, we need to pay more attention to the following algorithm development trends:
- From a hardware perspective, the accelerators of Domain Specific Architectures (DSA) [12] enable the third-wave AI algorithms to operate in a hybrid ecosystem consisting of Edge, Core, and Cloud. run anywhere in the system . Specifically, accelerators for specific domain architectures include the following examples: Nvidia's GPU, Xilinx's FPGA, Google's TPU, and artificial intelligence acceleration chips such as BrainChip's Akida Neural Processer, GraphCore's Intelligent Processing Unit (IPU), Cambrian's Machine Learning Unit (MLU) and more. These types of domain-specific architecture accelerators will be integrated into more information devices, architectures, and ecosystems by requiring less training data and being able to operate at lower power when needed. In response to this trend, the area where we need to focus on development is to develop a unified heterogeneous architecture approach that enables information systems to easily integrate and configure various different types of domain-specific architecture hardware accelerators. For Dell Technologies, we can leverage Dell's vast global supply chain and sales network to attract domain-specific architecture accelerator suppliers to adhere to the standard interfaces defined by Dell to achieve a unified heterogeneous architecture .
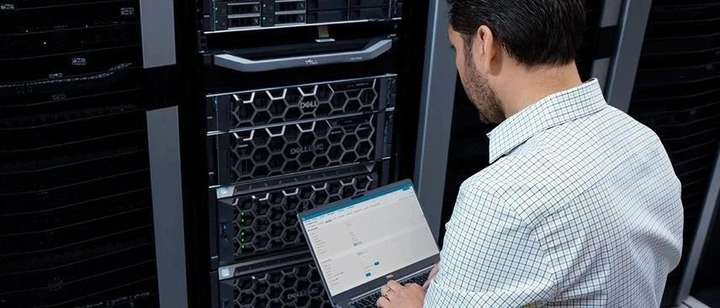
In addition to the above-mentioned development of algorithms and hardware that drives the “third wave of artificial intelligence”, another development direction that requires more attention is artificial intelligence automation (AutoML) [12]. As mentioned above, the development of artificial intelligence is becoming more and more complex, and for ordinary users, the professional skills threshold for using artificial intelligence is getting higher and higher. We urgently need to provide a complete set of information system architecture solutions that " make artificial intelligence simple ".
- We need to better operate and manage AI workloads, driving the simplification and optimization of information system architectures. Within the entire software stack of AI applications, we need to define " Easy Buttons " for future AI workloads . Specifically, we have the following technical directions to focus on:
- Develop a more simple and easy-to-use common API (Application Protocol Interface) for the advanced artificial intelligence algorithm framework , so that the information system architecture can integrate and use more advanced and complex algorithms.
- For artificial intelligence algorithms, we need to provide machine learning model parameters adaptive (Adaptive) selection and tuning (Tuning) strategies , according to the needs of users, automatically select the most suitable algorithm, and optimize the parameters of the algorithm to achieve the best performance.
- For the artificial intelligence data processing process (Pipeline), we need to establish the functions of process tracking, analysis and reuse , such as MLOps (Machine Learning Operation) described in [14]. Machine learning process management (MLOps) is the practice of creating new machine learning (ML) and deep learning (DL) models and deploying them into production through repeatable, automated workflows. When we have new artificial intelligence application problems, we can learn from the existing data processing process, and after a little analysis and modification, we can reuse the more mature artificial intelligence software and hardware solutions to meet new needs, thereby reducing repeated development. waste of resources.
- When our artificial intelligence system is deployed, our algorithm model still needs to have the evolution function of self-update, self-learning, and self-tuning . According to the changes of inference recognition scenarios and inference recognition tasks and the attenuation of algorithm accuracy, we use edge and cloud information system architecture to fully mobilize different computing resources to update, optimize and deploy our algorithm models. In the process of updating and deploying artificial intelligence models, we also use the latest algorithms such as model compression [17], data distillation [19], and knowledge distillation [18], so as to make full use of limited computing resources.
- We need to consider integrating the above AI-enabled automation services in multi-cloud and hybrid cloud environments, in line with Data Management and Orchestration, to create a complete and intelligent AI service platform .
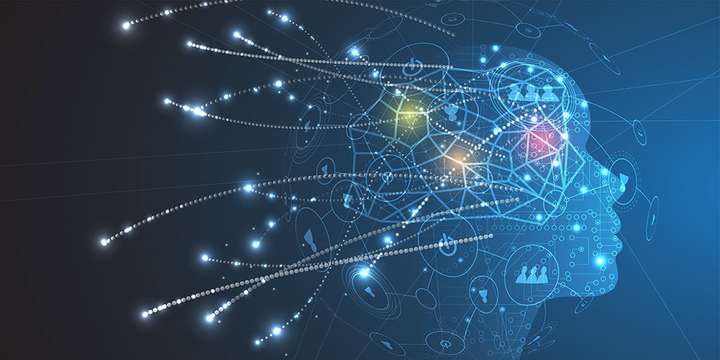
Technological innovation at Dell Technologies never stops. Our mission is to promote the progress of human society, promote technological innovation, and become the most important technology company in the data age. Our AI solutions will help our clients free themselves from the current complex processes of large-scale data processing, analysis and insights (Insights). The Research Office of our Office of CTO is also actively exploring the aforementioned AI development directions. We are committed to helping our clients make better use of state-of-the-art information system architectures, understand their data efficiently and in a timely manner, and bring greater value to their commercial business innovations .
Acknowledgments: I would like to thank the artificial intelligence research team of Dell Technologies China Research Institute (Li Sanping, Ni Jiacheng, Chen Qiang, Wang Zijia, Yang Wenbin, etc.) for their excellent research in the field of artificial intelligence. Their work results strongly support the content of this article.