uiux
Regular
Adapting SRT’s M1 Hardware Portal for Navy Facility Health Monitoring and Prioritization
Deep Neural Networks (DNN) have become a critical component of tactical applications, assisting the warfighter in interpreting and making decisions from vast and disparate sources of data. Whether image, signal or text data, remotely sensed or scraped from the web, cooperatively collected or intercepted, DNNs are the go-to tool for rapid processing of this information to extract relevant features and enable the automated execution of downstream applications. Deployment of DNNs in data centers, ground stations and other locations with extensive power infrastructure has become commonplace but at the edge, where the tactical user operates, is very difficult. Secure, reliable, high bandwidth communications are a constrained resource for tactical applications which limits the ability to routed data collected at the edge back to a centralized processing location. Data must therefore be processed in real-time at the point of ingest which has its own challenges as almost all DNNs are developed to run on power hungry GPUs at wattages exceeding the practical capacity of solar power sources typically available at the edge. So what then is the future of advanced AI for the tactical end user where power and communications are in limited supply? Neuromorphic processors may provide the answer. Blue Ridge Envisioneering, Inc. (BRE) proposes the development of a systematic and methodical approach to deploying Deep Neural Network (DNN) architectures on neuromorphic hardware and evaluating their performance relative to a traditional GPU-based deployment. BRE will develop and document a process for benchmarking a DNN’ s performance on a standard GPU, converting it to run on near-commercially available neuromorphic hardware, training and evaluating model accuracy for a range of available bit quantizations, characterizing the trade between power consumption and the various bit quantizations, and characterizing the trade between throughput/latency and the various bit quantizations. This process will be demonstrated on a Deep Convolutional Neural Network trained to classify objects in SAR imagery from the Air Force Research Laboratory’s MSTAR open source dataset. The BrainChip Akida Event Domain Neural Processor development environment will be utilized for demonstration as it provides a simulated execution environment for running converted models under the discrete, low quantization constraints of neuromorphic hardware.
---
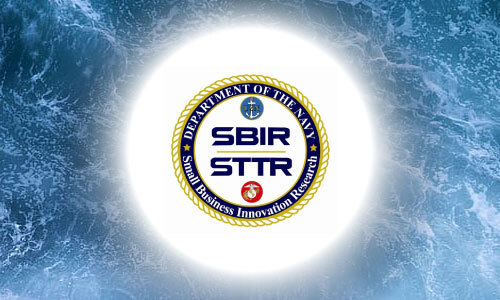
SRT Receives DoN Contract to Demonstrate the M1 Hardware Portal for Facility Health Monitoring to the Navy
SRT Labs is the recipient of a Department of Navy Small Business Innovative Research (SBIR) Phase I grant (SBIR 2020.1), as part of the Office of […]

SRT’s M1 is a cloud-based software framework that can integrate devices from different manufacturers into a single smart building ecosystem, analyzed and accessed through a single intelligence dashboard, so that data from one device can automate actions in another. Centralized control will provide ease of use for the end user, and all building health reporting happens in a single location. This commercial product was designed to be modular, in that hardware can be added or removed to scale the building’s ecosystem quickly, without requiring significant technical knowledge from the end-user. This stand-alone software tool will allow for a demonstration of: (1) data acquisition from integrated devices (IoT, IIoT, and logistics robots) across the facility, (2) management of historical device data, (3) alerting to off-nominal conditions from collected data, (4) cross-platform analytics that allow for information interpreted from more than one device, and (5) an interface for data display, reporting, and alerts.
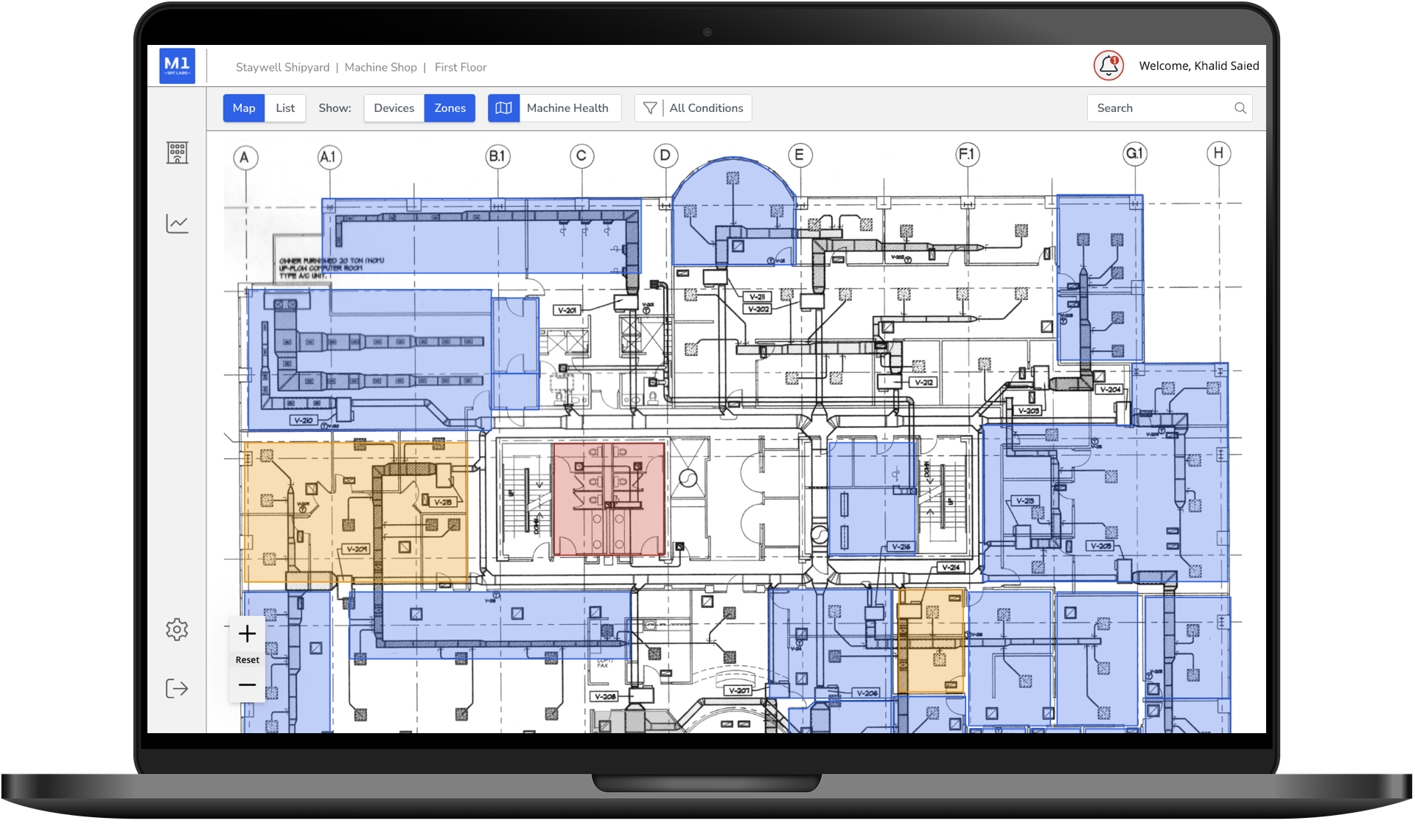
Service Robotics & Technologies | SRT Labs - Mission Control for Facilities
Intelligent technology hub that monitors and analyzes data to automate routines and reports in a platform that optimizes the environment

Monitor, Analyze, Automate
Our integration framework, the M1 Platform, brings together collaborative robotics and smart sensors with existing software and hardware to monitor, analyze and automate systems.
Last edited: